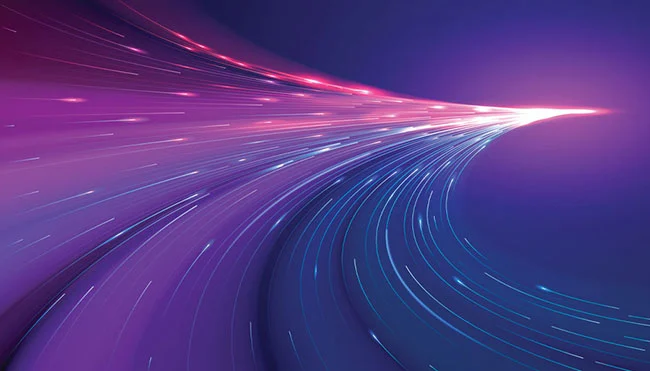
$$
\bar{\rho}{D}(t) \equiv e^{-\left(\mathcal{L}{x}+\mathcal{L}{p}\right) t} \tilde{\rho}{D}(t)
$$
to obtain
$$
\dot{\bar{\rho}}{D}=\overline{\mathcal{L}}{s p}(t) \bar{\rho}{D} $$ with the superoperator $$ \overline{\mathcal{L}}{s p}(t) \equiv e^{-\left(\mathcal{L}{n}+\mathcal{L}{p}\right) t} \mathcal{L}{s p} e^{\left(\mathcal{L}{s}+\mathcal{L}_{p}\right) t}
$$
taking the role of the interaction picture Liouvillian $(1 / i \hbar)\left[\tilde{H}{S R}(t), \cdot\right]$. To parallel , we have $$ \operatorname{tr}{p}\left[\bar{\rho}{D}(t)\right]=e^{-\mathcal{L}{n} t} \bar{\sigma}{-} a(t) \equiv \bar{\sigma}(t) $$ Then, after integratin once formally, substituting the integral form for $\bar{\rho}{D}(t)$ on the right-hand side of the equation, and taking the trace over the pump mode, we arrive at the equation of motion
$$
\dot{\bar{\sigma}}=\operatorname{tr}{p}\left[\overline{\mathcal{L}}{s p}(t) \rho_{D}(0)\right]+\int_{0}^{t} d t^{\prime} \operatorname{tr}{p}\left[\overline{\mathcal{L}}{s p}(t) \overline{\mathcal{L}}{s p}\left(t^{\prime}\right) \bar{\rho}{D}\left(t^{\prime}\right)\right]
$$
This equation is the analog.
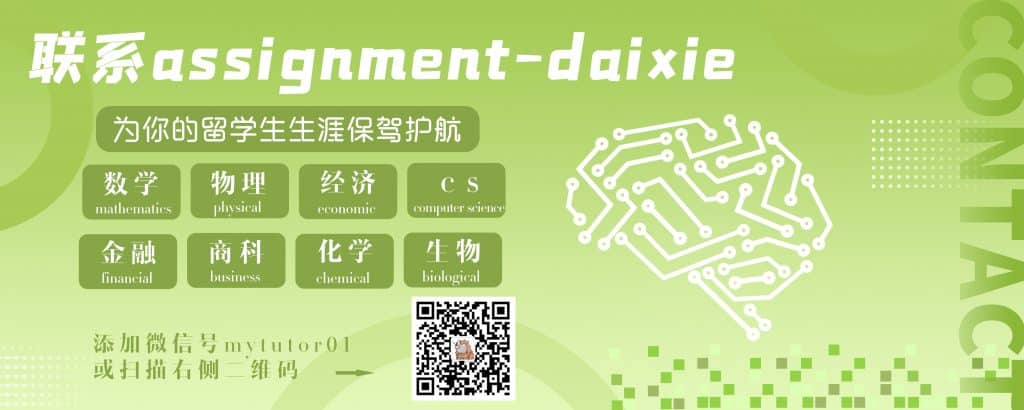
PHS1022 COURSE NOTES :
$$
q \equiv \tilde{\bar{\alpha}} / \tilde{\bar{\alpha}}{}, \quad p \equiv 1 / \tilde{\bar{\alpha}} \tilde{\bar{\alpha}}{},
$$
the equations to be solved are
$$
\begin{aligned}
&\frac{d q}{d \bar{t}}=\lambda\left(1-q^{2}\right) \
&\frac{d p}{d \bar{t}}=-p[\lambda(q+1 / q)-2]+2
\end{aligned}
$$
we have
$$
d q\left(\frac{1}{1+q}+\frac{1}{1-q}\right)=2 \lambda d \bar{t}
$$
whose direct integration gives the solution for $q$,
$$
q(\bar{t})=\frac{M(\bar{t})}{P(\bar{t})}
$$