这是一份uwa西澳大学PHYS1002的成功案例
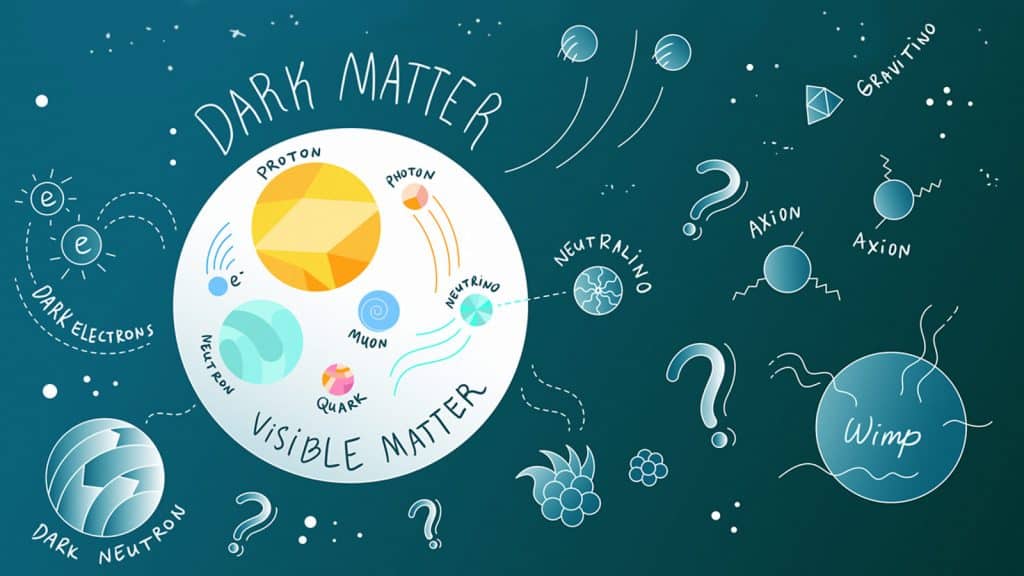
We claim that this equation, for a positive operator $\omega_{1}$ and $d^{2}$ unitaries $U_{x}$, implies that $\omega_{1}=d^{-1} \mathbb{I}$. To see this, expand the operator $A=|\phi\rangle\left\langle e_{k}\right| \omega_{1}^{-1}$ in the basis $U_{x}$ according to the formula $A=\sum_{x} U_{x} \operatorname{tr}\left(U_{x}^{} A \omega_{1}\right)$ : $$ \sum_{x}\left\langle e_{k}, U_{x}^{} \phi\right\rangle U_{x}=|\phi\rangle\left\langle e_{k}\right| \omega_{1}^{-1}
$$
Taking the matrix element $\left\langle\phi|\cdot| e_{k}\right\rangle$ of this equation and summing over $k$, we find
$$
\sum_{x, k}\left\langle e_{k}, U_{x}^{} \phi\right\rangle\left\langle\phi, U_{x} e_{k}\right\rangle=\sum_{x} \operatorname{tr}\left(U_{x}^{}|\phi\rangle\langle\phi| U_{x}\right)=d^{2}|\phi|^{2}=|\phi|^{2} \operatorname{tr}\left(\omega_{1}^{-1}\right)
$$
Hence $\operatorname{tr}\left(\omega_{1}^{-1}\right)=d^{2}=\sum_{k} r_{k}^{-1}$, where $r_{k}$ are the eigenvalues of $\omega_{1}$. Using again the fact that the smallest value of this sum under the constraint $\sum_{k} r_{k}=1$ is attained only for constant $r_{k}$, we find $\omega_{1}=d^{-1}$ I. and $\Omega$ is indeed maximally entangled.
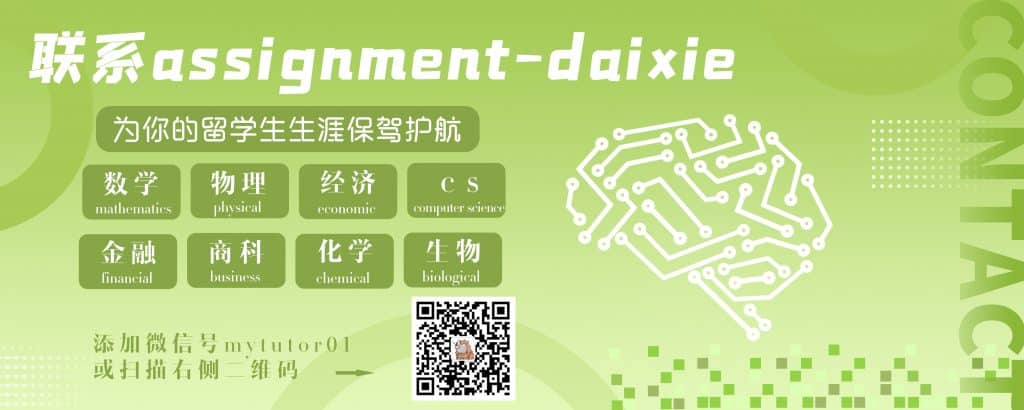
PHYS1002 COURSE NOTES :
Prepare the ground state
$$
\left|\varphi_{1}\right\rangle=|0 \ldots 0\rangle \otimes|0 \ldots 0\rangle
$$
in both quantum registers.
Achieve equal amplitude distribution in the first register, for instance by an application of a Hadamard transformation to each qubit:
$$
\left|\varphi_{2}\right\rangle=\frac{1}{\sqrt{2^{n}}} \sum_{x \in \mathbf{Z}{2}^{n}}|x\rangle \otimes|0 \ldots 0\rangle . $$ Apply $V{f}$ to compute $f$ in superposition. We obtain
$$
\left|\varphi_{3}\right\rangle=\frac{1}{\sqrt{2^{n}}} \sum_{x \in \mathbf{Z}^{n}}|x\rangle|f(x)\rangle .
$$