Multivariate distributions: expectation and variance-covariance matrix of a random vector; statement of properties of the bivariate and multivariate normal distribution.
这是一份Bath巴斯大学MA20227作业代写的成功案
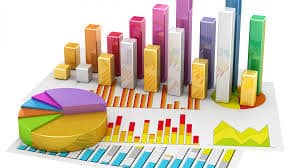
$$
G^{2}\left(M_{0}\right)=2 \sum_{i \in I} n_{i} \log \left(\frac{n_{i}}{\hat{m}{i}^{0}}\right) $$ where, for a cell $i$ belonging to the index set $I, n{i}$ is the frequency of observations in the $i$ th cell and $\hat{m}{i}^{0}$ are the expected frequencies for the considered model $M{0}$. For model comparison, two nested models $M_{0}$ and $M_{1}$ can be compared using the difference between their deviances:
$$
D=G_{0}^{2}-G_{1}^{2}=2 \sum_{i \in I} n_{i} \log \left(\frac{n_{i}}{\hat{m}{i}^{0}}\right)-2 \sum{i \in I} n_{i} \log \left(\frac{n_{i}}{\hat{m}{i}^{1}}\right)=2 \sum{i \in I} n_{i} \log \left(\frac{\hat{m}{i}^{1}}{\hat{m}{i}^{0}}\right)
$$
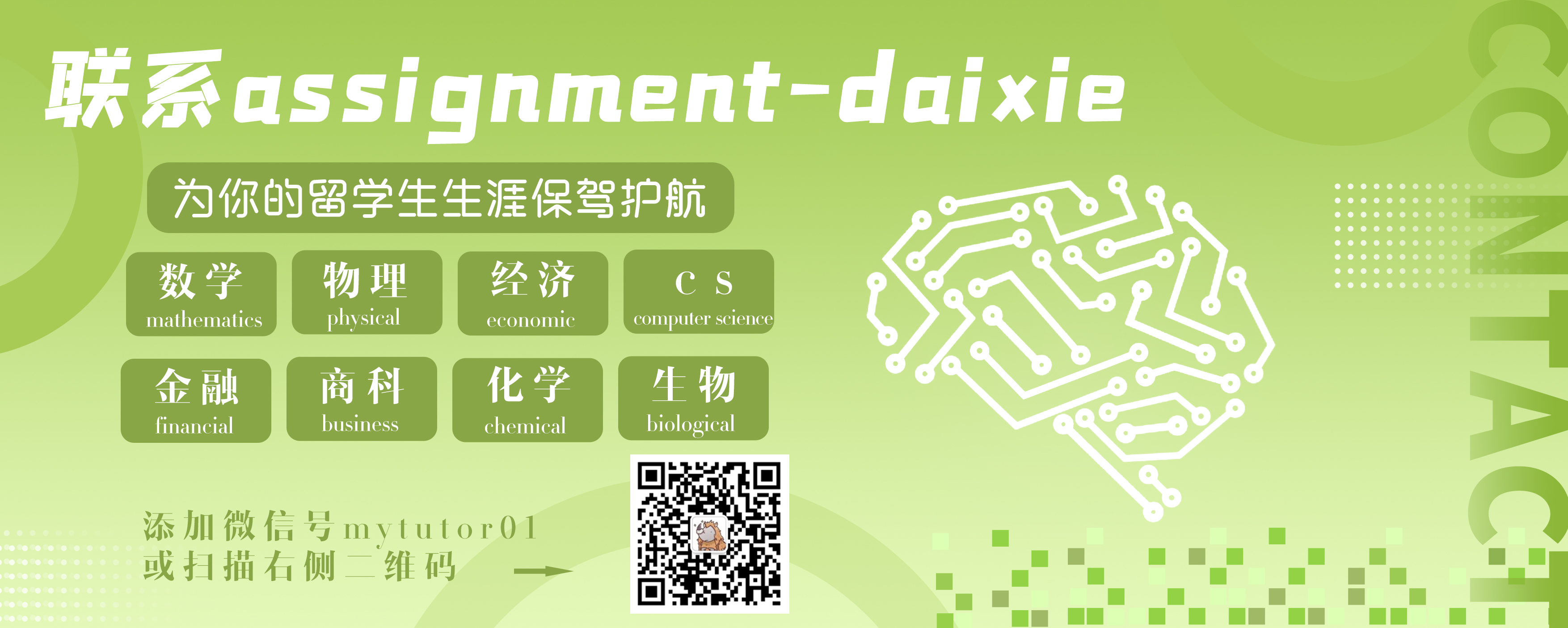
MA20227 COURSE NOTES :
If we knew $f$, the real model, we would be able to determine which of the approximating statistical models, different choices for $g$, will minimise the discrepancy. Therefore the discrepancy of $g$ (due to the parametric approximation) can be obtained as the discrepancy between the unknown probabilistic model and the best parametric statistical model, $p_{\theta_{0}}^{(I)}$ :
$$
\Delta\left(f, p_{\theta_{0}}^{(I)}\right)=\sum_{i=1}^{n}\left(f\left(x_{i}\right)-p_{\theta_{0}}^{(l)}\left(x_{i}\right)\right)^{2}
$$
However, since $f$ is unknown we cannot identify the best parametric statistical model. Therefore we will substitute $f$ with a sample estimate, be denoted by $p_{\hat{\theta}}^{(I)}(x)$, for which the $I$ parameters are estimated on the basis of the data. The discrepancy between this sample estimate of $f(x)$ and the best statistical model is called the discrepancy of $g$ (due to the estimation process):
$$
\Delta\left(p_{\hat{\theta}}^{(I)}, p_{\theta_{0}}^{(I)}\right)=\sum_{i=1}^{n}\left(p_{\hat{\theta}}^{(I)}\left(x_{i}\right)-p_{\theta_{0}}^{(I)}\left(x_{i}\right)\right)^{2}
$$