这是一份adelaide阿德莱德大学 STATS 3003 作业代写的成功案
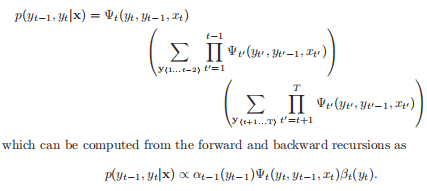
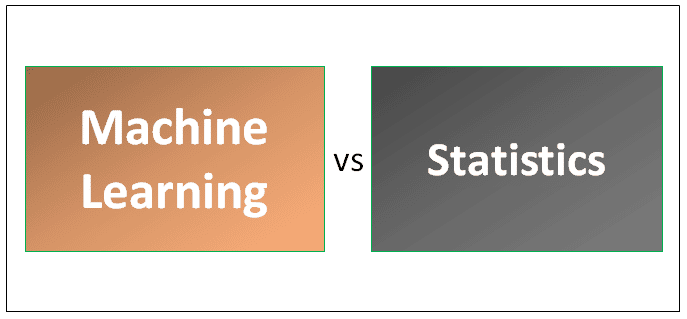
Proof
$$
\begin{aligned}
\Phi(G) &=\prod_{g \in G} \prod_{\theta \in \Theta_{g}} \phi_{g}\left(A_{g} \theta\right)=\prod_{g \in G} \prod_{\theta \in \Theta_{G}} \phi_{g}\left(A_{g} \theta\right)^{\left|\Theta_{g}\right| /\left|\Theta_{G}\right|} \
&=\prod_{\theta \in \Theta_{G}} \prod_{g \in G} \phi_{g}\left(A_{g} \theta\right)\left|\Theta_{g}\right| /\left|\Theta_{G}\right|=\prod_{\theta \in \Theta_{G}} \phi_{f s(G)}\left(A_{G} \theta\right)=\Phi(f s(G))
\end{aligned}
$$
While the above is correct, it is rather unnatural to have $e(D)$ and $e\left(D^{\prime}\right)$ be distinct atoms. If a set of logical variables has the same possible substitutions, like $D$ and $D^{\prime}$ here, we can do something better
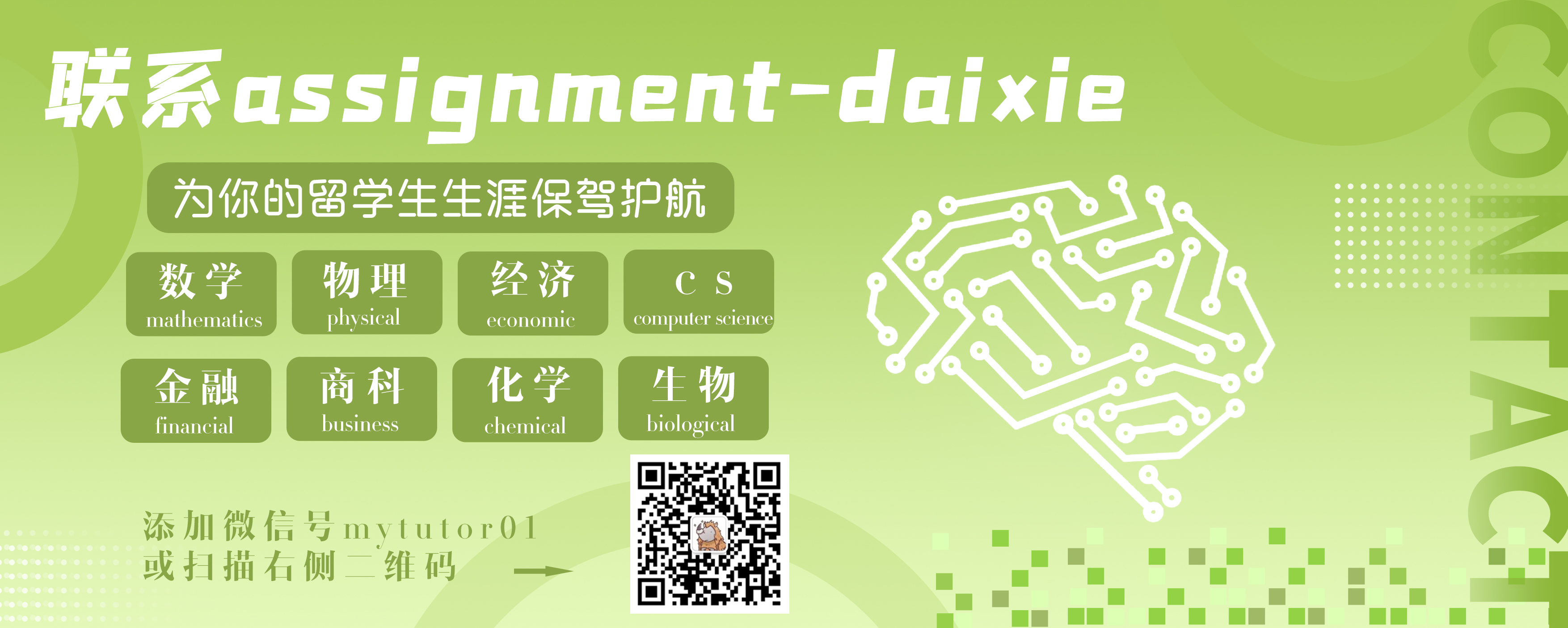
COMP SCI 3314 COURSE NOTES :
\begin{array}{r}
P\left(\boldsymbol{\theta}{\mathcal{G}}, \mathcal{D}\right)=P\left(\boldsymbol{\theta}{X}\right) L_{X}\left(\boldsymbol{\theta}{X}: \mathcal{D}\right) \ P\left(\boldsymbol{\theta}{Y \mid x^{1}}\right) \prod_{j: x^{j}=x^{1}} P\left(y^{j} \mid x^{n}: \boldsymbol{\theta}{Y \mid x^{1}}\right) \ P\left(\boldsymbol{\theta}{Y \mid x^{0}}\right) \prod_{j: x^{j}=x^{0}} P\left(y^{j} \mid x^{j}: \boldsymbol{\theta}_{Y \mid x^{0}}\right)
\end{array}