这是一份GLA格拉斯哥大学STATS1003_1作业代写的成功案例

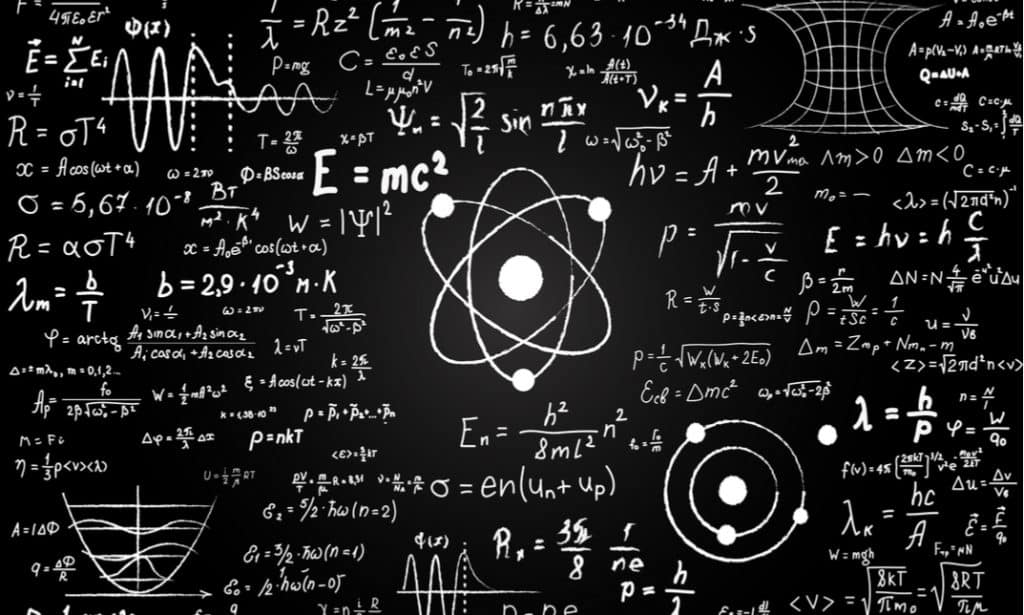
An appropriate model for a series with a single cycle is then
$$
y_{t}=A \cos (2 \pi f t \quad P) \quad u_{t}
$$
where $A$ is the amplitude and $P$ the phase of the cycle, and period $1 / f$, namely the number of time units from peak to peak. To allow for several $(r)$ frequencies operating simultaneously in the same data, the preceding may be generalised to
$$
y_{t}=\sum_{j=1}^{r} A_{j} \cos \left(2 \pi f_{j} t \quad P_{j}\right) \quad u_{t}
$$
For stationarity to apply, the $A_{j}$ may be taken as uncorrelated with mean 0 and the $P_{j}$ as uniform on $(0,2 \pi)$. Because
$$
\cos \left(2 \pi f_{j} t \quad P_{j}\right)=\cos \left(2 \pi f_{j} t\right) \cos \left(P_{j}\right)-\sin \left(2 \pi f_{j} t\right) \sin \left(P_{j}\right)
$$
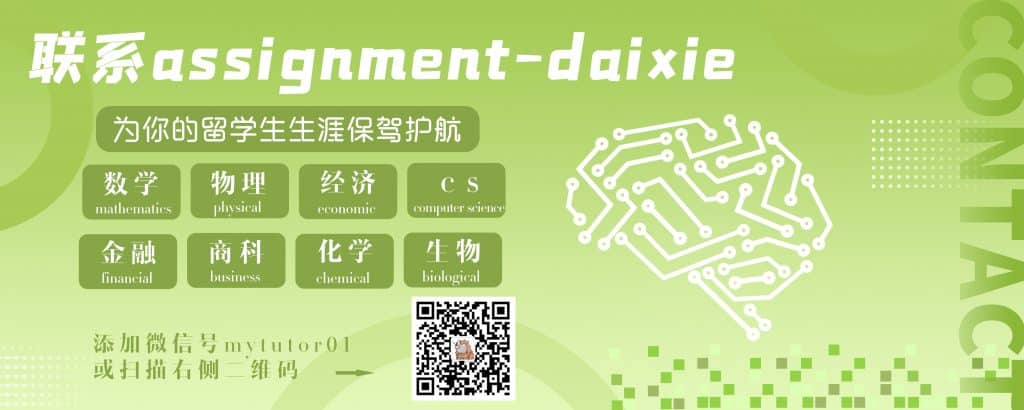
STATS1003_1 COURSE NOTES :
For discrete outcomes, dependence on past observations and predictors may be handled by adapting metric variable methods within the appropriate regression link. Thus for Poisson outcomes
$$
y_{t} \sim \operatorname{Poi}\left(\mu_{t}\right)
$$
an $\operatorname{AR}(1)$ dependence on previous values in the series could be specified
$$
\log \left(\mu_{t}\right)=\rho y_{t-1} \quad \beta x_{t}
$$
Here, non-stationarity or ‘explosive’ behaviour would be implied by $\rho>0$ (Fahrmeir and Tutz, 2001, p. 244), and in an MCMC framework stationarity would be assessed by the proportion of iterations for which $\rho$ was positive. Autoregressive errors lead to specification such as
$$
\log \left(\mu_{t}\right)=\beta x_{t} \quad \varepsilon_{t}
$$
with
$$
\varepsilon_{l}=\gamma \varepsilon_{t-1} \quad u_{l}
$$
for $t>1$, and $u_{t}$ being white noise.