这是一份andrews圣安德鲁斯大学 MT1007作业代写的成功案例


$$
P_{j}=\sum_{i=1}^{n}\left(x_{i} w_{i j}-\theta_{j}\right)
$$
To simplify the expression for potential, the bias term can be absorbed by adding a further input with constant value $x_{0}=1$, connected to the neuron $j$ through a weight $w_{0 j}=-\theta_{j}$ :
$$
P_{j}=\sum_{i=0}^{n}\left(x_{i} w_{i j}\right)
$$
Now consider the output signal. The output of the $j$ th neuron, $y_{j}$, is obtained by applying the activation function to potential $P_{j}$ :
$$
y_{j}=f\left(x, w_{j}\right)=f\left(P_{j}\right)=f\left(\sum_{i=0}^{n} x_{i} w_{i j}\right)
$$
The quantities in bold italics are vectors. In defining a neural network model, the activation function is typically one of the elements to specify. Three types are commonly employed: linear, stepwise and sigmoidal. A linear activation function is defined by
$$
f\left(P_{j}\right)=\alpha+\beta P_{j}
$$
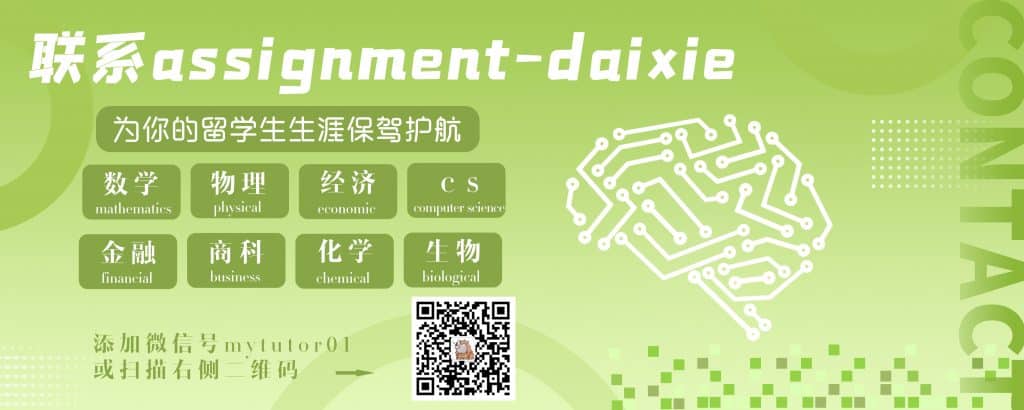
MT1007 COURSE NOTES :
$$
\bar{X}=\frac{1}{n} \sum_{i=1}^{n} X_{i}
$$
is always an unbiased estimator of the unknown population mean $\mu$, as it can be shown that $E(\bar{X})=\mu$. On the other hand, the sample variance
$$
S^{2}=\frac{1}{n} \sum_{i=1}^{n}\left(X_{i}-\bar{X}\right)^{2}
$$
is a biased estimator of the sample variance $\sigma^{2}$, as $E\left(S^{2}\right)=\frac{n^{-1}(n-1) \sigma^{2}}{n}$. Its bias is therefore
$$
\operatorname{bias}\left(S^{2}\right)=-\frac{1}{n} \sigma^{2}
$$