

For the Normal linear model
$$
\mathrm{E}\left(Y_{i}\right)=\mu_{i}=\mathbf{x}{i}^{T} \beta ; \quad Y{i} \sim \mathrm{N}\left(\mu_{i}, \sigma^{2}\right)
$$
for independent random variables $Y_{1}, \ldots, Y_{N}$, the deviance is
$$
D=\frac{1}{\sigma^{2}} \sum_{i=1}^{N}\left(y_{i}-\hat{\mu}{i}\right)^{2} $$ from equation (5.13). Let $\widehat{\mu}{i}(0)$ and $\widehat{\mu}{i}(1)$ denote the fitted values for model $M{0}$ (corresponding to null hypothesis $H_{0}$ ) and model $M_{1}$ (corresponding to the alternative hypothesis $H_{1}$ ), respectively. Then
$$
D_{0}=\frac{1}{\sigma^{2}} \sum_{i=1}^{N}\left[y_{i}-\widehat{\mu}{i}(0)\right]^{2} $$ and $$ D{1}=\frac{1}{\sigma^{2}} \sum_{i=1}^{N}\left[y_{i}-\widehat{\mu}_{i}(1)\right]^{2}
$$
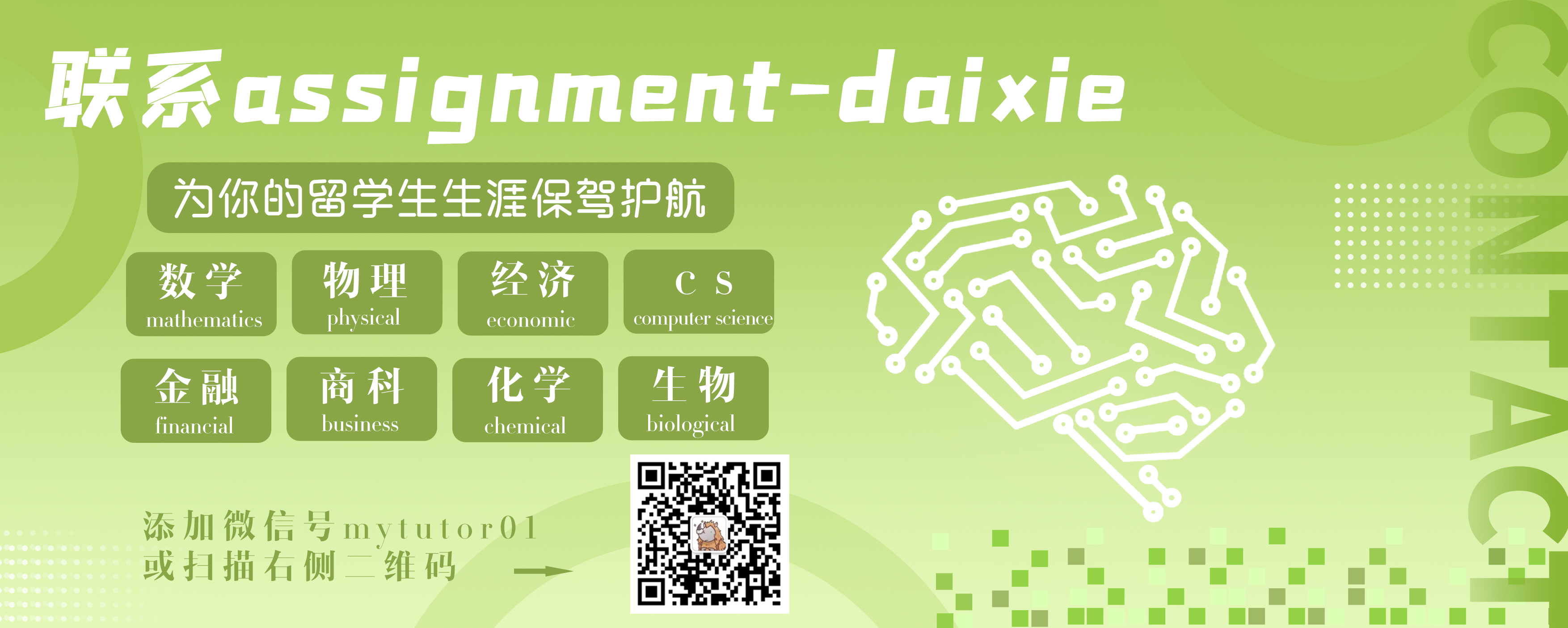
STAT0028 COURSE NOTES :
If $\mathrm{E}(\mathbf{y})=\mathbf{X} \boldsymbol{\beta}$ and $\mathrm{E}\left[(\mathbf{y}-\mathbf{X} \boldsymbol{\beta})(\mathbf{y}-\mathbf{X} \boldsymbol{\beta})^{T}\right]=\mathbf{V}$, where $\mathbf{V}$ is known, we can obtain the least squares estimator $\tilde{\beta}$ of $\beta$ without making any further assumptions about the distribution of $\mathbf{y}$. We minimize
$$
S_{w}=(\mathbf{y}-\mathbf{X} \boldsymbol{\beta})^{T} \mathbf{V}^{-1}(\mathbf{y}-\mathbf{X}) \boldsymbol{\beta}
$$
The solution of
$$
\frac{\partial S_{w}}{\partial \beta}=-2 \mathbf{X}^{T} \mathbf{V}^{-1}(\mathbf{y}-\mathbf{X} \boldsymbol{\beta})=0
$$
is
$$
\tilde{\boldsymbol{\beta}}=\left(\mathbf{X}^{T} \mathbf{V}^{-1} \mathbf{X}\right)^{-1} \mathbf{X}^{T} \mathbf{V}^{-1} \mathbf{y}
$$
provided the matrix inverses exist. In particular, for model (6.1), where the elements of $\mathbf{y}$ are independent and have a common variance then
$$
\tilde{\boldsymbol{\beta}}=\left(\mathbf{X}^{T} \mathbf{X}\right)^{-1} \mathbf{X}^{T} \mathbf{y} .
$$