Assignment-daixieTM为您提供佛罗里达大学University of Florida STA 4321 Mathematical Statistics 1数学统计代写代考和辅导服务!
Instructions:
Mathematical statistics is a branch of statistics that deals with the application of mathematical principles and methods to the analysis of data. It involves the development of mathematical models and techniques for analyzing data and making inferences about populations based on sample data.
Mathematical statistics is concerned with the study of probability theory, statistical inference, hypothesis testing, and decision theory. It is used in a wide variety of fields, including finance, economics, engineering, social sciences, and many other areas where data analysis is important.
Some of the key concepts in mathematical statistics include:
- Probability theory: Probability theory is the study of random events and their probabilities. It provides the foundation for statistical inference and hypothesis testing.
- Statistical inference: Statistical inference involves using sample data to make inferences about populations. This includes estimation of population parameters and hypothesis testing.
- Hypothesis testing: Hypothesis testing involves testing a hypothesis about a population using sample data. The null hypothesis is typically the hypothesis being tested, and the alternative hypothesis is the opposite of the null hypothesis.
- Decision theory: Decision theory involves making decisions based on statistical models and data. It includes methods for minimizing risk and maximizing utility.
Overall, mathematical statistics plays a critical role in helping researchers and practitioners make informed decisions based on data analysis.
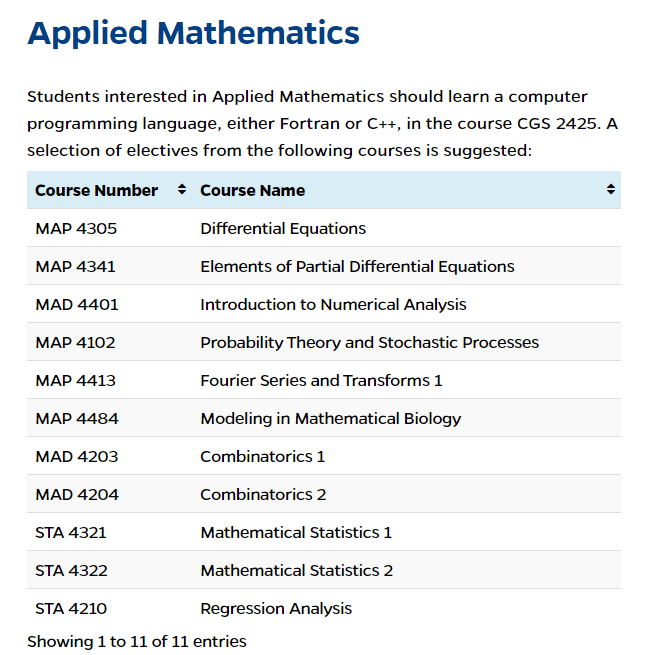
Let $X_1, \ldots, X_n$ be indpendent random variables with $$ X_i \sim P_{\theta_i}, \text { for } i=1, \ldots, n $$ (a). For $P_\theta=N(\theta, 1)$, determine the maximum likelihood estimate of $\left(\theta_1, \ldots, \theta_n\right)$ when there are no restrictions on the $\theta_i$.
$\begin{aligned} L\left(\theta_1, \ldots, \theta_n \mid x_1, \ldots, x_n\right) & =\prod_{i=1}^n f\left(x_i \mid \theta_i\right) \ & =\prod_{i=1}^n \frac{1}{\sqrt{2 \pi}} e^{-\frac{1}{2}\left(x_i-\theta_i\right)^2} .\end{aligned}$
Taking the logarithm of the likelihood function, we have
$\begin{aligned} \ell\left(\theta_1, \ldots, \theta_n \mid x_1, \ldots, x_n\right) & =\log L\left(\theta_1, \ldots, \theta_n \mid x_1, \ldots, x_n\right) \ & =-\frac{n}{2} \log 2 \pi-\frac{1}{2} \sum_{i=1}^n\left(x_i-\theta_i\right)^2 .\end{aligned}$
To find the maximum likelihood estimate of $\left(\theta_1,\ldots,\theta_n\right)$, we differentiate $\ell$ with respect to each $\theta_i$, set the derivatives equal to zero, and solve the resulting system of equations. Specifically,
$\frac{\partial \ell}{\partial \theta_i}=\sum_{j=1}^n\left(x_j-\theta_j\right) \delta_{i j}=x_i-\theta_i=0$
where $\delta_{ij}$ is the Kronecker delta. Thus, the maximum likelihood estimate of $\theta_i$ is $\hat{\theta}_i = x_i$, for $i=1,\ldots,n$.
(b). For $P_\theta=N(\theta, 1)$, determine the maximum likelihood estimate of $\theta$ when $\theta_1 = \cdots = \theta_n$.
When $\theta_1 = \cdots = \theta_n = \theta$, the likelihood function is given by
$L\left(\theta \mid x_1, \ldots, x_n\right)=\prod_{i=1}^n f\left(x_i \mid \theta\right)=\prod_{i=1}^n \frac{1}{\sqrt{2 \pi}} e^{-\frac{1}{2}\left(x_i-\theta\right)^2}$
Taking the logarithm of the likelihood function, we have
$\begin{aligned} \ell\left(\theta \mid x_1, \ldots, x_n\right) & =\log L\left(\theta \mid x_1, \ldots, x_n\right) \ & =-\frac{n}{2} \log 2 \pi-\frac{1}{2} \sum_{i=1}^n\left(x_i-\theta\right)^2 .\end{aligned}$
To find the maximum likelihood estimate of $\theta$, we differentiate $\ell$ with respect to $\theta$, set the derivative equal to zero, and solve for $\hat{\theta}$. Specifically,
$\frac{\partial \ell}{\partial \theta}=\sum_{i=1}^n\left(x_i-\theta\right)=n \bar{x}-n \theta=0$
where $\bar{x} = \frac{1}{n} \sum_{i=1}^n x_i$ is the sample mean. Thus, the maximum likelihood estimate of $\theta$ is $\hat{\theta} = \bar{x}$.
Let $X$ and $Y$ be the numbers of wins in 4 and 8 games respectively. For 4 games there are $2^4=16$ equally likely outcomes e.g. $W L W W$ which has 3 wins so $X=3$. Using our basic counting principles there will be $\left(\begin{array}{l}4 \ j\end{array}\right)$ outcomes containing $j$ wins and so $P(X=3)=4 \times 0.5^4=0.25$.
Similarly with 8 games there are $2^8=256$ equally likely outcomes and this time $P(Y=5)=56 \times 0.5^8=$ $0.2188$ so the former is larger.
For part (b) remember that $X \geq 3$ means all the outcomes with at least 3 wins out of 4 etc and that we sum probabilities over mutually exclusive outcomes. Doing the calculations, $P(X \geq 3)=$ $0.25+0.0625=0.3125$ is less than $P(Y \geq 5)=0.2188+0.1094+0.0313+0.0039=0.3633-$ we deduce from this that the chance of a drawn series falls as the series gets longer.
A lucky dip at a school fête contains 100 packages of which 40 contain tickets for prizes. Let $X$ denote the number of prizes you win when you draw out three of the packages. Find the probability density of $X$ i.e. $P(X=i)$ for each appropriate $i$.
There are $\left(\begin{array}{c}100 \ 3\end{array}\right)$ choices of three packages (in any ordering). There are $\left(\begin{array}{c}60 \ 3\end{array}\right)$ choices of three packages without prizes. Hence $P(X=0)=\left(\begin{array}{c}60 \ 3\end{array}\right) /\left(\begin{array}{c}100 \ 3\end{array}\right) \approx 0.2116$. If a single prize is won this can happen in $\left(\begin{array}{c}40 \ 1\end{array}\right) \cdot\left(\begin{array}{c}60 \ 2\end{array}\right)$ ways. Hence $P(X=1)=\left(\begin{array}{c}40 \ 1\end{array}\right) \cdot\left(\begin{array}{c}60 \ 2\end{array}\right) /\left(\begin{array}{c}100 \ 3\end{array}\right) \approx 0.4378$ and similarly $P(X=2)=\left(\begin{array}{c}40 \ 2\end{array}\right)$. $\left(\begin{array}{c}60 \ 1\end{array}\right) /\left(\begin{array}{c}100 \ 3\end{array}\right) \approx 0.2894$ and $P(X=3)=\left(\begin{array}{c}40 \ 3\end{array}\right) /\left(\begin{array}{c}100 \ 3\end{array}\right) \approx 0.0611$ (there is some small rounding error in the given values).
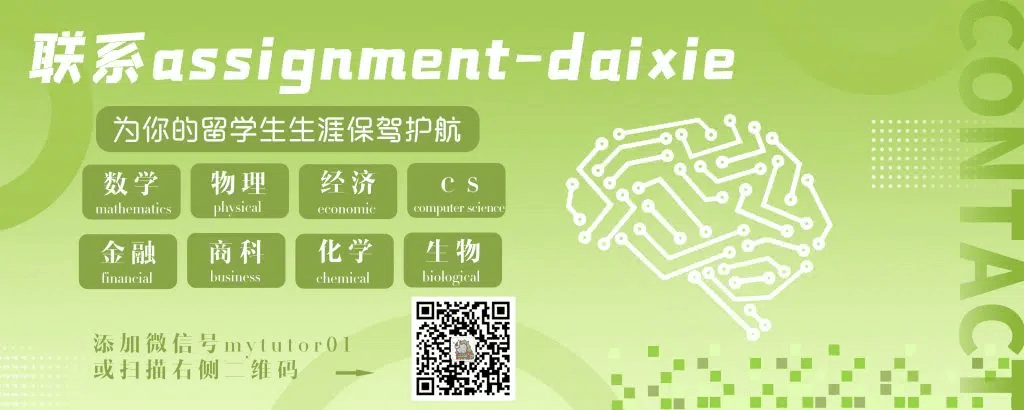