这是一份GLA格拉斯哥大学MATHS2025_1/MATHS3016_1作业代写的成功案例
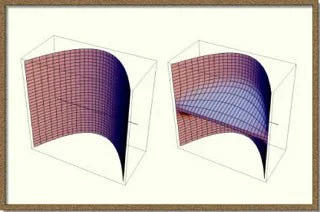
Proof of (b) is easy:
$$
d\left(y_{n}, x\right) \leq d\left(y_{n}, x_{n}\right)+d\left(x_{n}, x\right) \rightarrow 0 .
$$
(c) is also easy. Look at
$$
d\left(x_{n}, y_{n}\right) \leq d\left(x_{n}, x_{n}^{\prime}\right)+d\left(x_{n}^{\prime}, y_{n}^{\prime}\right)+d\left(y_{n}^{\prime}, y_{n}\right) .
$$
Taking limits, we get $\lim {n} d\left(x{n}, y_{n}\right) \leq \lim {n} d\left(x{n}^{\prime}, y_{n}^{\prime}\right)$. Similar argument shows the other way inequality and hence the proof.
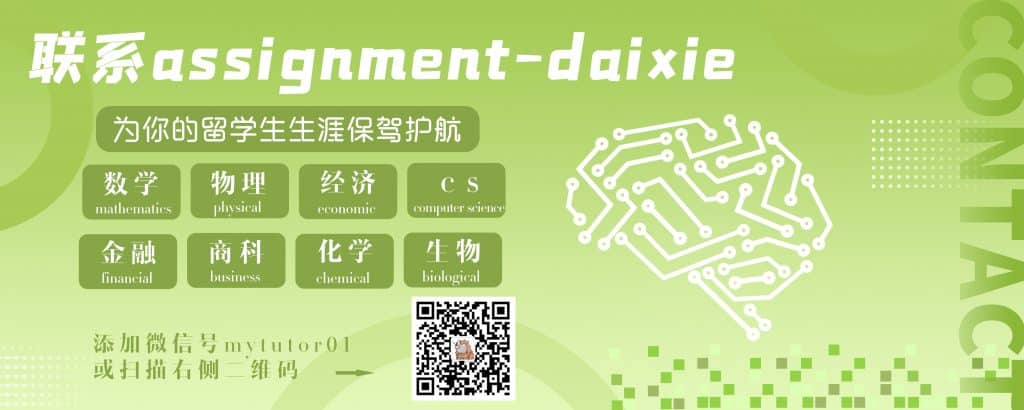
MATHS5039_1 COURSE NOTES :
For, if $\varepsilon>0$ is given, by the Cauchy nature of $\left(x_{n}\right)$, there exists $N \in \mathbb{N}$ such that $d\left(x_{m}, x_{n}\right)<\varepsilon$ for $m, n \geq N$. We have, for $n \geq N$,
$$
d\left(\tilde{x}{n}, \xi\right):=\lim {m \rightarrow \infty} d\left(x_{n}, x_{m}\right)<\varepsilon .
$$
It is clear that the map $\varphi: X \rightarrow \tilde{X}$ given by $\varphi(x)=\tilde{x}$ is an isometry. $d(\varphi(x), \varphi(y)):=\lim _{n} d(x, y)$, the limit of a constant sequence.
We claim that the image $\varphi(X)$ of $X$ under the map $\varphi: x \mapsto \tilde{x}$ is dense