这是一份应用回归分析作业代写的成功案
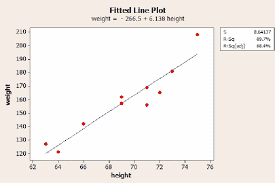
$$
\hat{\boldsymbol{\beta}}{O L S}=\frac{n}{n-1} \hat{\boldsymbol{\Sigma}}{\boldsymbol{x}}^{-1} \hat{\boldsymbol{\Sigma}}{\boldsymbol{x} Y} \stackrel{D}{\rightarrow} \boldsymbol{\beta}{O L S} \text { as } \mathrm{n} \rightarrow \infty
$$
and
$$
\hat{\boldsymbol{\Sigma}} \boldsymbol{x} Y=\frac{1}{n} \sum_{i=1}^{n} \boldsymbol{x}{i} Y{i}-\overline{\boldsymbol{x}} \bar{Y}
$$
Thus
$$
\hat{\Sigma}{\boldsymbol{x} Y}=\frac{1}{n}\left[\sum{j: Y_{j}=1} \boldsymbol{x}{j}(1)+\sum{j: Y_{j}=0} \boldsymbol{x}{j}(0)\right]-\overline{\boldsymbol{x}} \hat{\pi}{1}=
$$
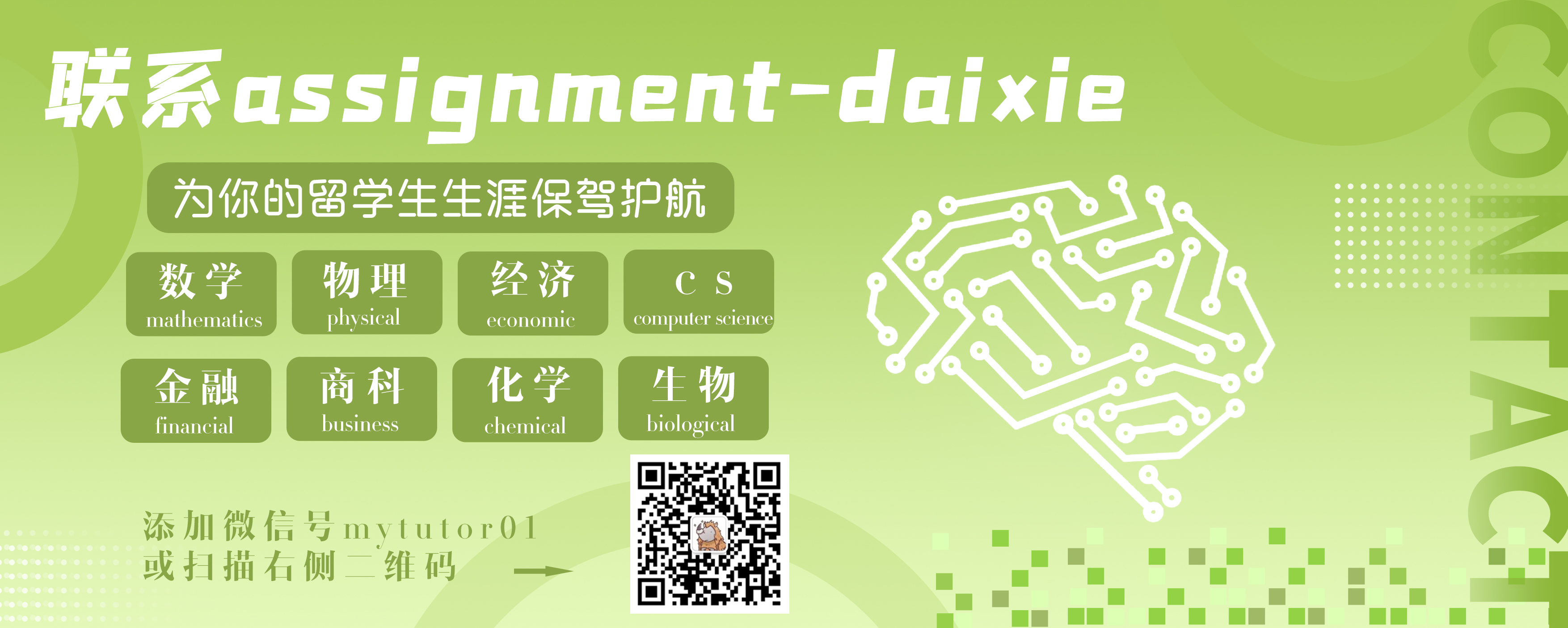
MTH 412/NURS 629/STAT 4530/STA 111L/QTM 100/STA 290 COURSE NOTES :
The discriminant function estimator
$$
\hat{\boldsymbol{\beta}}{D}=\frac{n(n-1)}{N{0} N_{1}} \hat{\Sigma}^{-1} \hat{\Sigma}{\boldsymbol{x}} \hat{\boldsymbol{\beta}}{O L S} .
$$
Now when the conditions of Definition $10.3$ are met and if $\mu_{1}-\mu_{0}$ is small enough so that there is not perfect classification, then
$$
\boldsymbol{\beta}{L R}=\Sigma^{-1}\left(\mu{1}-\mu_{0}\right) .
$$
Empirically, the OLS ESP and LR ESP are highly correlated for many LR data sets where the conditions are not met, eg when some of the predictors are factors. This suggests that $\boldsymbol{\beta}{L R} \approx d \boldsymbol{\Sigma}{\boldsymbol{x}}^{-1}\left(\boldsymbol{\mu}{1}-\boldsymbol{\mu}{0}\right)$ for many LR data sets where $d$ is some constant depending on the data. Results from Haggstrom (1983) suggest that if a binary regression model is fit using OLS software for MLR, then a rough approximation is $\hat{\boldsymbol{\beta}}{L R} \approx \hat{\boldsymbol{\beta}}{O L S} / M S E$. So a rough approximation is LR ESP $\approx(\mathrm{OLS}$ ESP $) / M S E$.