这是一份GLA格拉斯哥大MATHS5077_1作业代写的成功案例
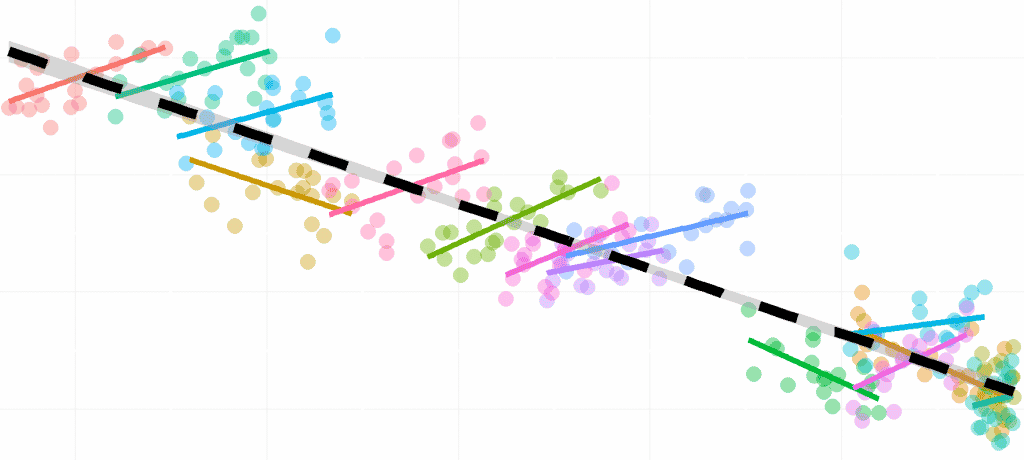
The methods discussed above for the full parameter vector now directly carry over to calculate local influences on the geometric surface defined by $\mathrm{LD}{1}(\omega)$. We now partition $\ddot{L}$ as $$ \ddot{L}=\left(\begin{array}{ll} \ddot{L}{11} & \ddot{L}{12} \ \ddot{L}{21} & \ddot{L}{22} \end{array}\right), $$ according to the dimensions of $\theta{1}$ and $\theta_{2}$. Cook (1986) has then shown that the local influence on the estimation of $\theta_{1}$, of perturbing the model in the direction of a normalized vector $\boldsymbol{h}$, is given by
$$
C_{h}\left(\boldsymbol{\theta}{1}\right)=2\left|\boldsymbol{h}^{\prime} \Delta^{\prime}\left[\ddot{L}^{-1}-\left(\begin{array}{cc} 0 & 0 \ 0 & \ddot{L}{22}^{-1}
\end{array}\right)\right] \Delta \boldsymbol{h}\right|
$$
Because all eigenvalues of the malrix
$$
\left(\begin{array}{ll}
\ddot{L}{11} & \ddot{L}{12} \
\ddot{L}{21} & \ddot{L}{22}
\end{array}\right)\left(\begin{array}{cc}
0 & 0 \
0 & \ddot{L}{22}^{-1} \end{array}\right)=\left(\begin{array}{cc} 0 & \ddot{L}{12} \ddot{L}_{22}^{-1} \
0 & I
\end{array}\right)
$$
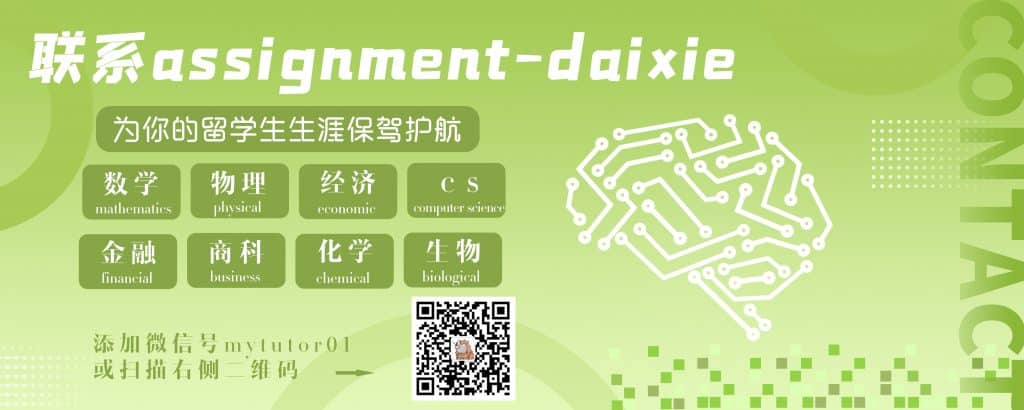
MATHS5077_1 COURSE NOTES :
A measure of influence, proposed , is then
$$
\rho_{i}=-\left(\hat{\boldsymbol{\theta}}-\hat{\boldsymbol{\theta}}{(i)}^{1}\right)^{\prime} \ddot{L}\left(\hat{\boldsymbol{\theta}}-\hat{\boldsymbol{\theta}}{(i)}^{1}\right)
$$$$
\boldsymbol{\Delta}{i}=-\sum{j \neq i} \boldsymbol{\Delta}{j}=\ddot{L}{(i)}(\widehat{\boldsymbol{\theta}})\left(\hat{\boldsymbol{\theta}}{(i)}^{1}-\widehat{\boldsymbol{\theta}}\right) $$ such that expression (11.4) becomes $$ C{i}=-2\left(\hat{\boldsymbol{\theta}}-\hat{\boldsymbol{\theta}}{(i)}^{1}\right)^{\prime} \ddot{L}{(i)} \ddot{L}^{-1} \ddot{L}{(i)}\left(\hat{\boldsymbol{\theta}}-\hat{\boldsymbol{\theta}}{(i)}^{1}\right)
$$