Assignment-daixieTM为您提供东英吉利大学University of East Anglia ECO-7001B APPLIED ECONOMETRICS应用计量经济学代写代考和辅导服务!
Instructions:
Applied econometrics is the application of statistical and mathematical methods to analyze economic data. Econometrics is a branch of economics that uses statistical methods to test economic theories and forecast economic trends. Applied econometrics applies these techniques to real-world data sets to answer questions related to economic policy, market analysis, and business decisions.
Applied econometrics involves the use of mathematical and statistical models to analyze economic data. These models can be used to test economic theories, estimate the impact of policy changes, and forecast economic outcomes. Econometricians use a variety of statistical techniques, including regression analysis, time-series analysis, and panel data analysis, to analyze economic data.
Applied econometrics is used in a wide range of fields, including finance, marketing, public policy, and international trade. For example, an econometrician might use data on consumer behavior to estimate the impact of a new advertising campaign on sales. Or, they might use data on economic growth to forecast the impact of a new trade agreement.
Overall, applied econometrics provides a valuable tool for economists and other researchers to analyze economic data and answer important questions about the economy.
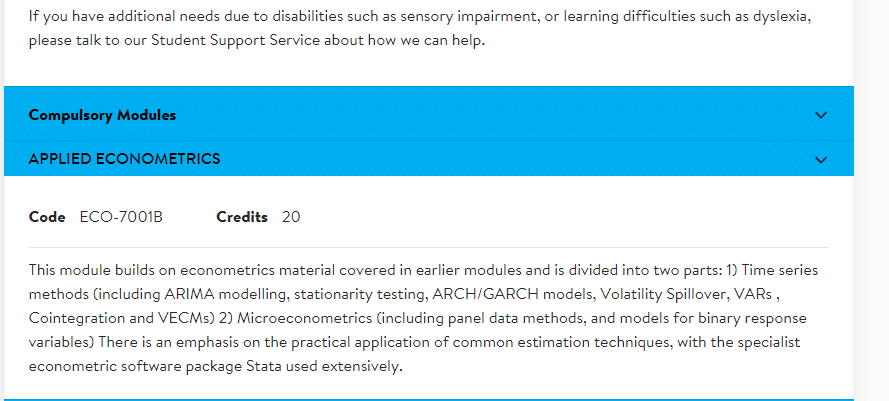
Consider $E[Y \mid X]$ where $X$ is a dummy variable that equals one with probability $p$ and is zero otherwise. Prove that the CEF and the regression of $\mathrm{Y}$ on $\mathrm{X}$ are the same in this case. Do this by showing that for Bernoulli $\mathrm{X}$ : $$ \begin{aligned} & \alpha=\mathrm{E}(\mathrm{Y})-\beta \mathrm{E}(\mathrm{X})=\mathrm{E}[\mathrm{Y} \mid \mathrm{X}=0] \\ & \beta=\mathrm{COV}(\mathrm{X}, \mathrm{Y}) / \mathrm{V}(\mathrm{X})=(\mathrm{E}[\mathrm{Y} \mid \mathrm{X}=1]-\mathrm{E}[\mathrm{Y} \mid \mathrm{X}=0]) \end{aligned} $$
First, we note that for a Bernoulli $X$, we have $\mathrm{E}(X) = p$ and $\mathrm{Var}(X) = p(1-p)$.
The conditional expectation of $Y$ given $X$ is given by:
\mathrm{E}(Y \mid X)= \begin{cases}\mathrm{E}(Y \mid X=1) & \text { if } X=1 \ \mathrm{E}(Y \mid X=0) & \text { if } X=0\end{cases}
We can write the conditional expectation of $Y$ as a linear function of $X$ as follows:
$\mathrm{E}(Y \mid X)=\alpha+\beta X$
where $\alpha = \mathrm{E}(Y) – \beta \mathrm{E}(X)$ and $\beta = \mathrm{Cov}(X, Y)/\mathrm{Var}(X)$.
To see that the CEF and the regression of $Y$ on $X$ are the same, we need to show that $\alpha = \mathrm{E}(Y \mid X=0)$ and $\beta = \mathrm{E}(Y \mid X=1) – \mathrm{E}(Y \mid X=0)$.
To see that $\alpha = \mathrm{E}(Y \mid X=0)$, we note that when $X=0$, the conditional expectation of $Y$ is given by $\mathrm{E}(Y \mid X=0)$. Therefore, we have:
$\alpha+\beta \times 0=\mathrm{E}(Y \mid X=0)$
which implies that $\alpha = \mathrm{E}(Y \mid X=0)$.
To see that $\beta = \mathrm{E}(Y \mid X=1) – \mathrm{E}(Y \mid X=0)$, we note that:
$\begin{aligned} & \mathrm{E}(Y \mid X=1)=\alpha+\beta \times 1 \ & \mathrm{E}(Y \mid X=0)=\alpha+\beta \times 0\end{aligned}$
Subtracting the second equation from the first, we obtain:
$\mathrm{E}(Y \mid X=1)-\mathrm{E}(Y \mid X=0)=\beta \times 1-\beta \times 0=\beta$
which implies that $\beta = \mathrm{E}(Y \mid X=1) – \mathrm{E}(Y \mid X=0)$.
Thus, we have shown that the CEF and the regression of $Y$ on $X$ are the same for a Bernoulli $X$.
Re-estimate the model in 2a, allowing the relationship between wages and schooling to differ by race.
Construct an F-test to test the null hypothesis that the returns to an additional year of schooling are the
same for all races.
To estimate the relationship between wages and schooling by race, we can add an interaction term between race and schooling in the regression model in 2a. The model can be written as follows:
$\ln ($ wage $)=\beta 0+\beta 1$ educ $+\beta 2$ black $+\beta 3^{\star}$ (educ*black $)+\varepsilon$
where black is an indicator variable equal to 1 if the person is black and 0 otherwise, and ε is the error term.
To test the null hypothesis that the returns to an additional year of schooling are the same for all races, we can construct an F-test for the joint significance of the interaction term (educ*black) and the black dummy variable. The null hypothesis is that β3 = 0, which implies that the returns to an additional year of schooling are the same for blacks and non-blacks. The alternative hypothesis is that β3 is not equal to zero, which implies that the returns to an additional year of schooling differ by race.
To conduct the F-test, we first estimate the restricted model, which does not include the interaction term:
$\ln ($ wage $)=\beta 0+\beta 1$ educ $+\beta$ bblack $+\varepsilon$
We then estimate the unrestricted model, which includes the interaction term:
$\ln ($ wage $)=\beta 0+\beta 1$ educ $+\beta$ 2black $+\beta 3^{\star}($ educ*black $)+\varepsilon$
We can then calculate the F-statistic using the following formula:
$F=((R S S R-R S S U R) / q) /(R S S U R /(n-k-1))$
where RSSR is the residual sum of squares from the restricted model, RSSUR is the residual sum of squares from the unrestricted model, q is the number of restrictions (in this case, q = 1), n is the sample size, and k is the number of parameters estimated in the unrestricted model.
If the calculated F-value is greater than the critical value of the F-distribution with q and n-k-1 degrees of freedom at the desired significance level, we reject the null hypothesis and conclude that the returns to an additional year of schooling differ by race.
Note that this F-test assumes that the error variance is constant across race groups. If this assumption is violated, the test may not be valid and alternative methods may need to be used.
Re-estimate the model in 2a, with a full set of interactions between race and sex. How many new
variables do you need to add to your regression? Using your coefficients, calculate the expected log
hourly wage in each race-sex category for a 25-year-old worker with 12 years of schooling.
To estimate a model with a full set of interactions between race and sex, we need to add six new variables:
- Black_female
- Black_male
- Hispanic_female
- Hispanic_male
- Other_female
- Other_male
The regression model is:
$log(wage) = \beta_0 + \beta_1 educ + \beta_2 female + \beta_3 black + \beta_4 hispanic + \beta_5 other + \beta_6 female \times black + \beta_7 female \times hispanic + \beta_8 female \times other + \beta_9 black \times hispanic + \beta_{10} black \times other + \beta_{11} hispanic \times other + \epsilon$
where:
- educ: years of education
- female: 1 if female, 0 if male
- black: 1 if black, 0 otherwise
- hispanic: 1 if Hispanic, 0 otherwise
- other: 1 if other race, 0 otherwise
To calculate the expected log hourly wage for a 25-year-old worker with 12 years of schooling in each race-sex category, we can use the following formulas:
- Black female: $\hat{y} = \beta_0 + \beta_1(12) + \beta_2(1) + \beta_3(1) + \beta_6(1) = (\beta_0 + \beta_3 + \beta_6) + \beta_1(12) + \beta_2$
- Black male: $\hat{y} = \beta_0 + \beta_1(12) + \beta_3(1) = (\beta_0 + \beta_3) + \beta_1(12)$
- Hispanic female: $\hat{y} = \beta_0 + \beta_1(12) + \beta_2(1) + \beta_4(1) + \beta_7(1) = (\beta_0 + \beta_4 + \beta_7) + \beta_1(12) + \beta_2$
- Hispanic male: $\hat{y} = \beta_0 + \beta_1(12) + \beta_4(1) = (\beta_0 + \beta_4) + \beta_1(12)$
- Other female: $\hat{y} = \beta_0 + \beta_1(12) + \beta_2(1) + \beta_5(1) + \beta_8(1) = (\beta_0 + \beta_5 + \beta_8) + \beta_1(12) + \beta_2$
- Other male: $\hat{y} = \beta_0 + \beta_1(12) + \beta_5(1) = (\beta_0 + \beta_5) + \beta_1(12)$
Note that in each formula, we use the coefficients obtained from the regression model with full interactions. We plug in the value of 12 for educ and 1 for the relevant race-sex indicator variables.
We cannot provide exact values for these expected log hourly wages without the coefficients from the regression model, but this is the general process for calculating them.
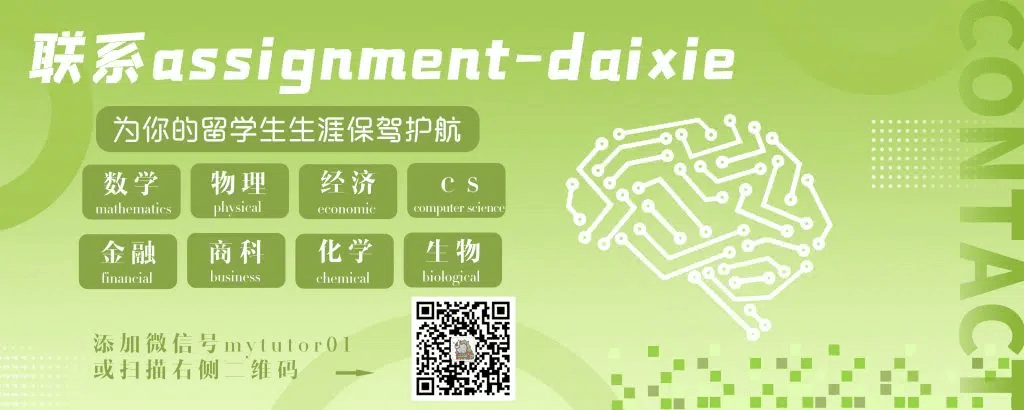