这是一份liverpool利物浦大学ECON121的成功案例
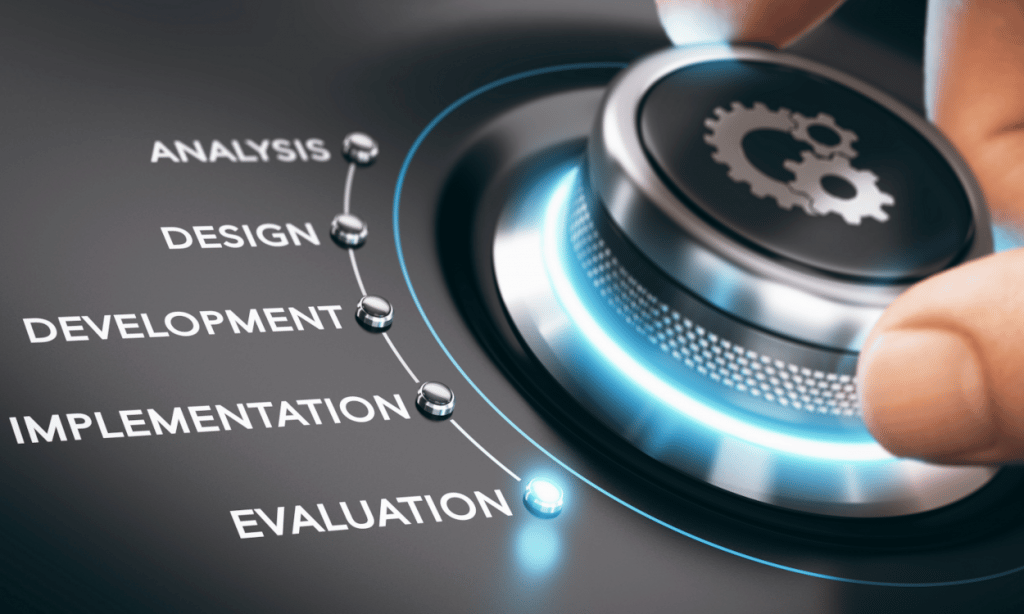
- $\theta=\lim {n \rightarrow \infty} \bar{y}{n}$ must exist, and the marginal distribution (given $\theta$ ) for each of the $y_{i}$ must be $p\left(y_{i} \mid \theta\right)=$ Bernoulli $(\theta)=\theta^{y_{i}}(1-\theta)^{1-y_{i}}$;
- $H(t)=\lim {n \rightarrow \infty} P\left(y{n} \leq t\right)$, the limiting cumulative distribution function (CDF) of the $\bar{y}{n}$ values, must also exist for all $t$ and must be a valid CDF, where $P$ is Your joint probability distribution on $\left(y{1}, y_{2}, \ldots\right)$; and
- Your predictive distribution for the first $n$ observations can be expressed as
$$
p\left(y_{1}, \ldots, y_{n}\right)=\int_{0}^{1} \prod_{i=1}^{n} \theta^{\gamma_{i}}(1-\theta)^{1-y_{i}} d H(\theta) .
$$
When (as will essentially always be the case in realistic applications) Your joint distribution $P$ is sufficiently regular that $H$ possesses a density (with respect to Lebesgue measure), $d H(\theta)=p(\theta) d \theta,(1)$ can be written in a more accessible way as
$$
p\left(y_{1}, \ldots, y_{n}\right)=\int_{0}^{1} \theta^{s_{n}}(1-\theta)^{n-s_{n}} p(\theta) d \theta
$$
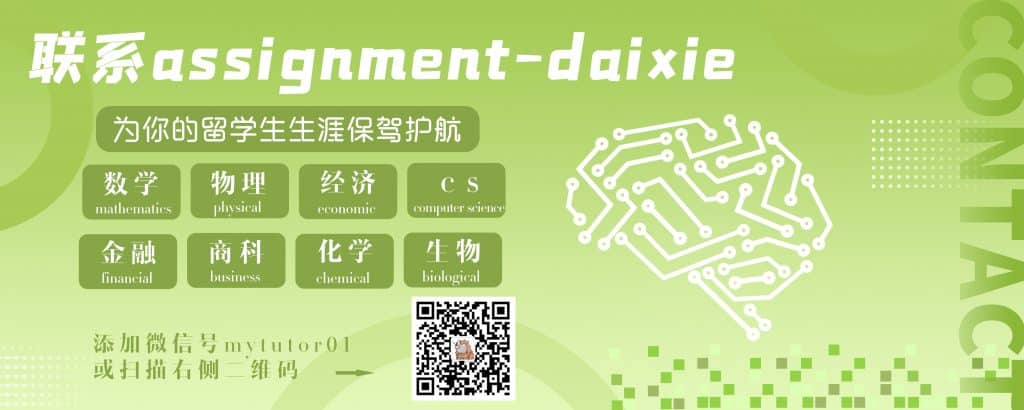
ECON121 COURSE NOTES :
The random effects model implies a homoskedastic variance var $\left(u_{i t}\right)=\sigma_{\mu}^{2}+\sigma_{\mathrm{v}}^{2}$ for all $i$ and $t$, and an equi-correlated block-diagonal covariance matrix which exhibits serial correlation over time only between the disturbances of the same individual. In fact,
$$
\begin{aligned}
\operatorname{cov}\left(u_{i t}, u_{j s}\right) &=\sigma_{\mu}^{2}+\sigma_{v}^{2} & \text { for } & i=j, t=s \
&=\sigma_{\mu}^{2} & & \text { for } i=j, t \neq s
\end{aligned}
$$
and zero otherwise. This also means that the correlation coefficient between $u_{i t}$ and $u_{j s}$ is
$$
\begin{aligned}
\rho &=\operatorname{correl}\left(u_{i t}, u_{j s}\right)=1 & & \text { for } i=j, t=s \
&=\sigma_{\mu}^{2} /\left(\sigma_{\mu}^{2}+\sigma_{\mathrm{v}}^{2}\right) & & \text { for } i=j, t \neq s
\end{aligned}
$$