这是一份贝叶斯统计推断作业代写的成功案
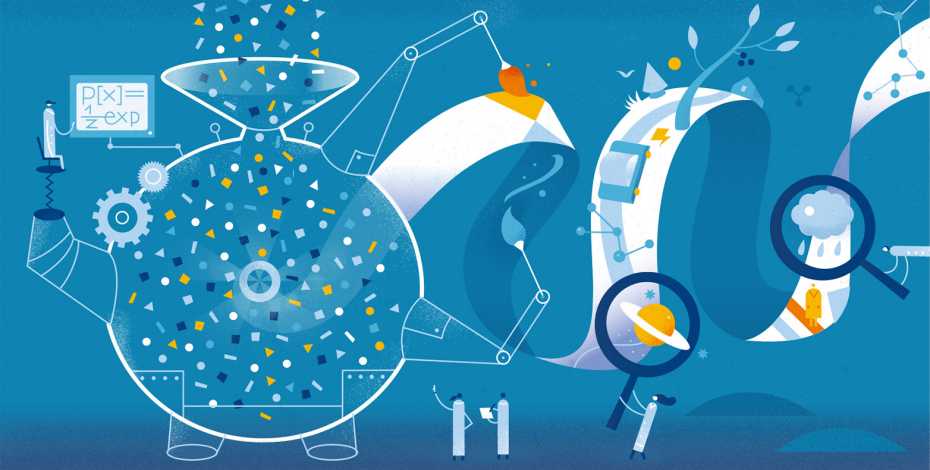
Keep in mind that the leading terms here for large $k$ are the last two and, in fact, at $k=n$, they cancel each other so that our argument does not prove the fallacious statement for $c \geq 1$ that there is no connected component of size $n$, since there is. Let
$$
f(k)=\ln n+k+k \ln \ln n-2 \ln k+k \ln c-c k \ln n+c k^{2} \frac{\ln n}{n}
$$
Differentiating with respect to $k$,
$$
f^{\prime}(k)=1+\ln \ln n-\frac{2}{k}+\ln c-c \ln n+\frac{2 c k \ln n}{n}
$$
and
$$
f^{\prime \prime}(k)=\frac{2}{k^{2}}+\frac{2 c \ln n}{n}>0 .
$$
Thus, the function $f(k)$ attains its maximum over the range $[2, n / 2]$ at one of the extreme points 2 or $n / 2$. At $k=2, f(2) \approx(1-2 c) \ln n$ and at $k=n / 2, f(n / 2) \approx-c \frac{n}{4} \ln n$. So
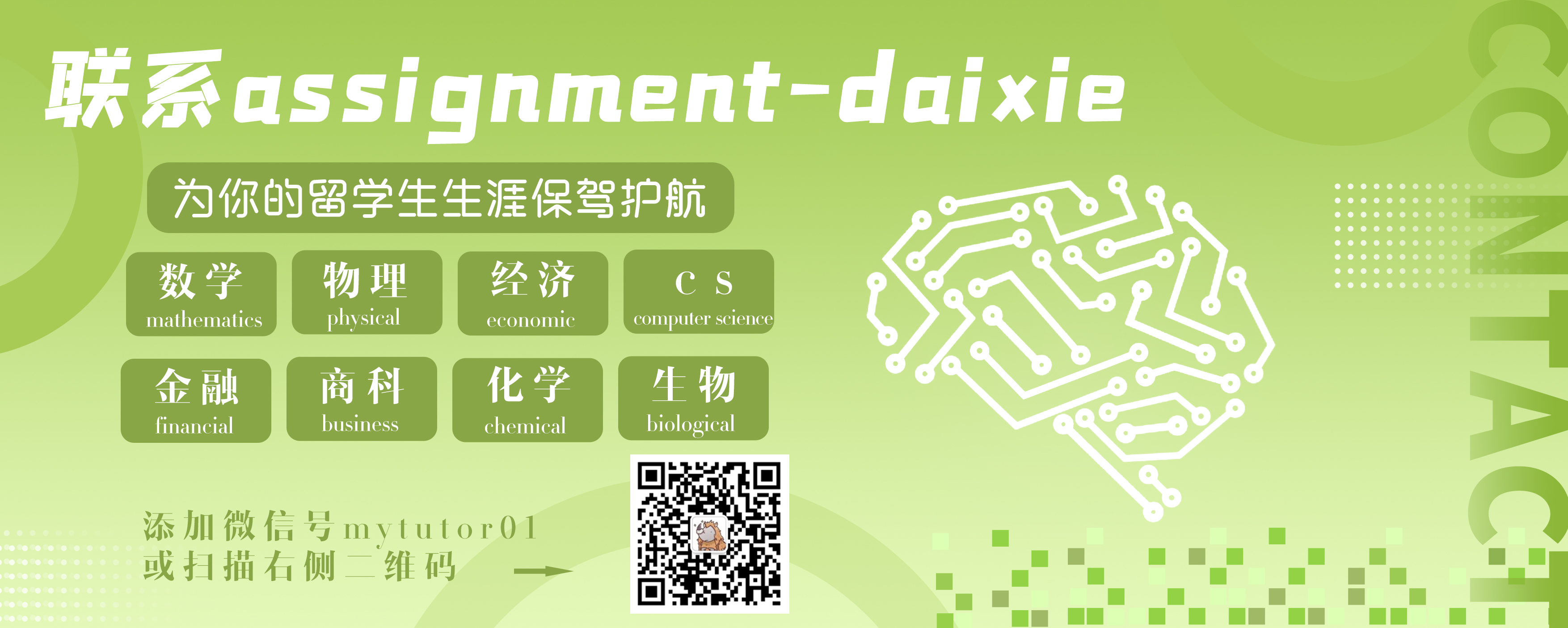
DATA1001/ COMP615/CS910/MATHS 7027 COURSE NOTES :
$$
\sum_{i=0}^{\infty} \frac{i}{i !}=\sum_{i=1}^{\infty} \frac{i}{i !}=\sum_{i=1}^{\infty} \frac{1}{(i-1) !}=\sum_{i=0}^{\infty} \frac{1}{i !}
$$
and
$$
\sum_{i=0}^{\infty} \frac{i^{2}}{i !}=\sum_{i=1}^{\infty} \frac{i}{(i-1) !}=\sum_{i=0}^{\infty} \frac{i+1}{i !}=\sum_{i=0}^{\infty} \frac{i}{i !}+\sum_{i=0}^{\infty} \frac{1}{i !}=2 \sum_{i=0}^{\infty} \frac{1}{i !}
$$
Thus,
$$
\sum_{i=0}^{\infty} \frac{i(i-2)}{i !}=\sum_{i=0}^{\infty} \frac{i^{2}}{i !}-2 \sum_{i=0}^{\infty} \frac{i}{i !}=0
$$