这是一份liverpool利物浦大学MATH346的成功案例
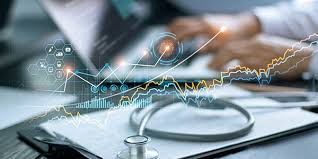
$$
\varphi \sim \operatorname{Beta}\left(a_{0}, b_{0}\right),
$$
with density
$$
p(\varphi)=\frac{1}{\mathrm{~B}\left(a_{0}, b_{0}\right)} \varphi^{a_{0}-1}(1-\varphi)^{b_{0}-1}
$$
The advantage is that the posterior is also a Beta distribution (hence the name conjugate), with density
$$
p(\varphi \mid \text { data }) \propto \ell(\varphi \mid \text { data }) \times p(\varphi) \propto \varphi^{a_{0}+a-1}(1-\varphi)^{b_{0}+b-1}
$$
The prior weights $a_{0}$ and $b_{0}$ are added to the observed counts $a_{1}$ and $b_{1}$, so that at the interim analysis
$$
\varphi \mid \text { data } \sim \varphi \mid a_{1} \sim \operatorname{Beta}\left(a_{1}+a_{0}, b_{1}+b_{0}\right) .
$$
The predictive distribution, which is a mixture of Binomial distributions, is naturally called a Beta-Binomial distribution
$$
a_{2} \mid a_{1} \sim \operatorname{Beta}-\operatorname{Bin}\left(a_{1}+a_{0}, b_{1}+b_{0} ; n_{2}\right) .
$$
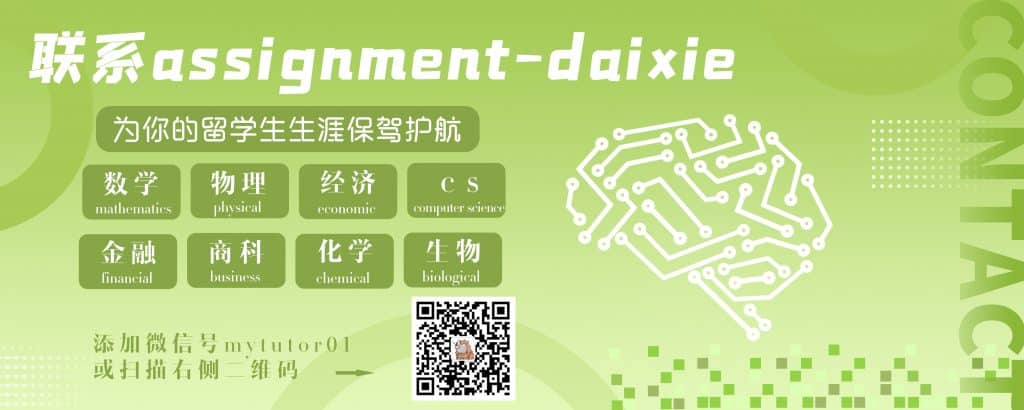
ECON364 COURSE NOTES :
$$
\delta(\mathbf{x})=\frac{\partial E[y \mid \mathbf{x}]}{\partial \mathbf{x}}=\exp \left(\boldsymbol{\beta}^{\prime} \mathbf{x}\right) \times \boldsymbol{\beta}
$$
As in any regression model, this measure is a function of the data point at which it is evaluated. For analysis of the Poisson model, researchers typically use one of the two approaches: The marginal effects, computed at the mean, or the center of the data are
$$
\delta(\overline{\mathbf{x}})=\frac{\partial E[y \mid \overline{\mathbf{x}}]}{\partial \overline{\mathbf{x}}}=\exp \left(\boldsymbol{\beta}^{\prime} \overline{\mathbf{x}}\right) \times \boldsymbol{\beta},
$$
where $\overline{\mathbf{x}}=(1 / N) \sum_{i=1}^{N} \mathbf{x}{i}$ is the sample mean of the data. An alternative, commonly used measure is the set of average partial effects, $$ \bar{\delta}(\mathbf{X})=\frac{1}{N} \sum{i=1}^{N} \frac{\partial E\left[y \mid \mathbf{x}{i}\right]}{\partial \mathbf{x}{i}}=\frac{1}{N} \sum_{i=1}^{N} \exp \left(\boldsymbol{\beta}^{\prime} \mathbf{x}_{i}\right) \times \boldsymbol{\beta} .
$$