Assignment-daixieTM为您提供布里斯托大学University of Bristol Probability, Statistics and Econometrics EFIM10024概率、统计和计量经济学代写代考和辅导服务!
Instructions:
Probability, statistics, and econometrics are three closely related fields that are fundamental to understanding the behavior of systems that involve uncertainty, such as financial markets, natural phenomena, and social systems.
Probability theory deals with the study of random events and the likelihood of their occurrence. It is concerned with the formulation and manipulation of mathematical models that can be used to quantify the likelihood of different outcomes in a given situation.
Statistics is the science of collecting, analyzing, and interpreting data. It involves the use of mathematical and computational tools to summarize and make inferences from data, and to test hypotheses about the underlying mechanisms that generate the data.
Econometrics is the application of statistical methods to the analysis of economic data. It is concerned with developing models that can be used to understand the relationships between different economic variables and to make predictions about future economic outcomes.
All three fields are essential for making informed decisions in a variety of contexts, such as business, finance, public policy, and scientific research. They provide a rigorous framework for understanding and quantifying uncertainty, and for making predictions based on available data.
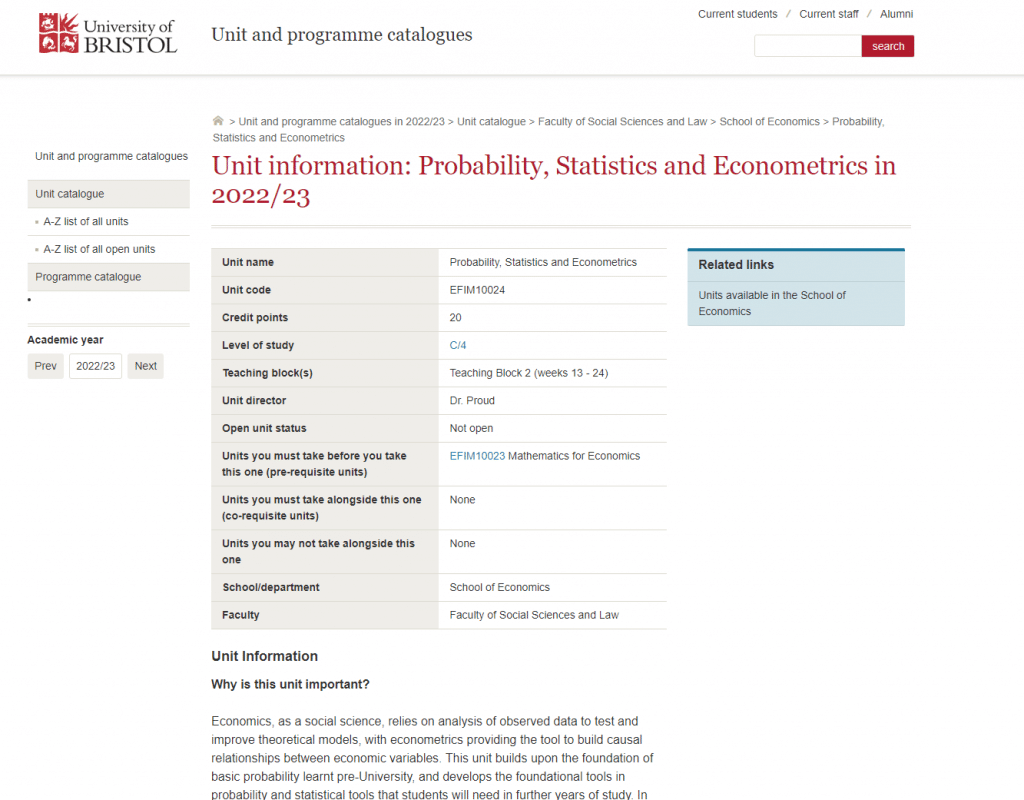
(Multivariate limit theorems) Let $\mathbf{X}=\left(X_1, \ldots, X_m\right)^{\prime}$ and $\mathbf{X}_n=\left(X_{n 1}, \ldots, X_{n m}\right)^{\prime}$ be $m$-dimensional random vectors. Define a norm $\|\mathbf{X}\|=\sqrt{X_1^2+\ldots+X_m^2}$. (a) Show that $E\|\mathbf{X}\|<\infty$ if and only if $E\left|X_i\right|<\infty$ for all $i=1, \ldots, m$.
(a) By the Cauchy-Schwarz inequality, we have
$|\mathbf{X}|=\sqrt{X_1^2+\ldots+X_m^2} \leq \sqrt{m \cdot\left(X_1^2+\ldots+X_m^2\right)}=\sqrt{m} \cdot|\mathbf{X}|_2$,
where $|\cdot|_2$ denotes the Euclidean norm. Therefore, if $E|\mathbf{X}|<\infty$, then $E|\mathbf{X}|_2<\infty$. Since $|X|_2 \leq \sqrt{m} \cdot |X|$, we have $E|X|<\infty$. Conversely, if $E\left|X_i\right|<\infty$ for all $i=1,\ldots,m$, then
$E|\mathbf{X}| \leq \sqrt{\sum_{i=1}^m E\left(X_i^2\right)} \leq \sqrt{m \cdot \max _{i=1, \ldots, m} E\left(X_i^2\right)}<\infty$.
(b) Define $\mathbf{X}_n \rightarrow^p \mathbf{X}$ if for any $\varepsilon>0, \lim _{n \rightarrow \infty} P\left\{\left\|\mathbf{X}_n-\mathbf{X}\right\|>\varepsilon\right\}=0$. Show $\mathbf{X}_n \rightarrow^p \mathbf{X}$ if and only if $X_{n i} \rightarrow^p X_i$ for all $i=1, \ldots, m$.
(b) Assume $\mathbf{X}_n \rightarrow^p \mathbf{X}$. Let $\epsilon > 0$ and let $i$ be any fixed index between $1$ and $m$. Then
$P\left(\left|X_{n i}-X_i\right|>\epsilon\right) \leq P\left(\left|\mathbf{X}_n-\mathbf{X}\right|>\epsilon\right) \rightarrow 0$
as $n\rightarrow\infty$. Thus, $X_{ni} \rightarrow^p X_i$ for all $i=1,\ldots,m$. Conversely, assume that $X_{ni} \rightarrow^p X_i$ for all $i=1,\ldots,m$. Let $\epsilon > 0$. Then
$P\left(\left|\mathbf{X}n-\mathbf{X}\right|>\epsilon\right)=P\left(\max {i=1, \ldots, m}\left|X_{n i}-X_i\right|>\frac{\epsilon}{\sqrt{m}}\right) \leq \sum_{i=1}^m P\left(\left|X_{n i}-X_i\right|>\frac{\epsilon}{\sqrt{m}}\right) \rightarrow 0$
as $n\rightarrow\infty$. Therefore, $\mathbf{X}_n \rightarrow^p \mathbf{X}$.
The multivariate Central Limit Theorem states that if $\mathbf{X}_1,\mathbf{X}_2,\ldots$ are independent random vectors with $\mathbb{E}(\mathbf{X}_n)=\boldsymbol{\mu}$ and $\text{Var}(\mathbf{X}_n)=\boldsymbol{\Sigma}$, and if $\boldsymbol{\Sigma}$ is positive definite, then
$\frac{\mathbf{S}_n-\boldsymbol{\mu}}{\sqrt{n}} \Rightarrow \mathcal{N}(\mathbf{0}, \mathbf{\Sigma})$
where $\mathbf{S}n=\sum{i=1}^n\mathbf{X}_i$ and $\Rightarrow$ denotes convergence in distribution.
To prove this, we can use a version of the one-dimensional Linderberg-Feller’s theorem for multivariate random vectors. Specifically, let $\mathbf{X}_1,\mathbf{X}_2,\ldots$ be independent random vectors with $\mathbb{E}(\mathbf{X}_n)=\boldsymbol{\mu}$ and $\text{Var}(\mathbf{X}_n)=\boldsymbol{\Sigma}$, and let $\boldsymbol{\Sigma}$ be positive definite. Suppose that for all $\epsilon>0$,
$\lim {n \rightarrow \infty} \frac{1}{s_n^2} \sum{i=1}^n \mathbb{E}\left(\left|\mathbf{X}i-\boldsymbol{\mu}\right|^2 \mathbf{1}{\left{\left|\mathbf{X}_i-\boldsymbol{\mu}\right|>\epsilon s_n\right}}\right)=0$,
$\lim {n \rightarrow \infty} \frac{1}{s_n^2} \sum{i=1}^n \mathbb{E}\left(\left|\mathbf{X}i-\boldsymbol{\mu}\right|^2 \mathbf{1}{\left{\left|\mathbf{X}_i-\boldsymbol{\mu}\right|>\epsilon s_n\right}}\right)=0$,
where $s_n^2=\sum_{i=1}^n\text{Var}(|\mathbf{X}_i-\boldsymbol{\mu}|)$. Then,
$\frac{\mathbf{S}_n-\boldsymbol{\mu}}{s_n} \Rightarrow \mathcal{N}(\mathbf{0}, \mathbf{I})$,
where $\mathbf{I}$ is the identity matrix.
To prove this, we can use characteristic functions. Let $\boldsymbol{\lambda}$ be a fixed $m$-dimensional vector with $|\boldsymbol{\lambda}|=1$. Then, \begin{align*} \mathbb{E}(\exp{i\boldsymbol{\lambda}^{\prime}(\mathbf{S}n-\boldsymbol{\mu})/s_n})&=\mathbb{E}(\exp{i\boldsymbol{\lambda}^{\prime}(\sum{i=1}^n(\mathbf{X}i-\boldsymbol{\mu}))/s_n})\ &=\prod{i=1}^n\mathbb{E}(\exp{i\boldsymbol{\lambda}^{\prime}(\mathbf{X}i-\boldsymbol{\mu})/s_n})\ &=\prod{i=1}^n\exp{-\frac{1}{2}|\boldsymbol{\lambda}|^2\text{Var}(|\mathbf{X}_i-\boldsymbol{\mu}|)/s_n^2+o(1/s_n^2)}\ &=\exp{-\frac{1}{2}|\boldsymbol{\lambda}|^2/n+o(1/n)}, \end{align*}
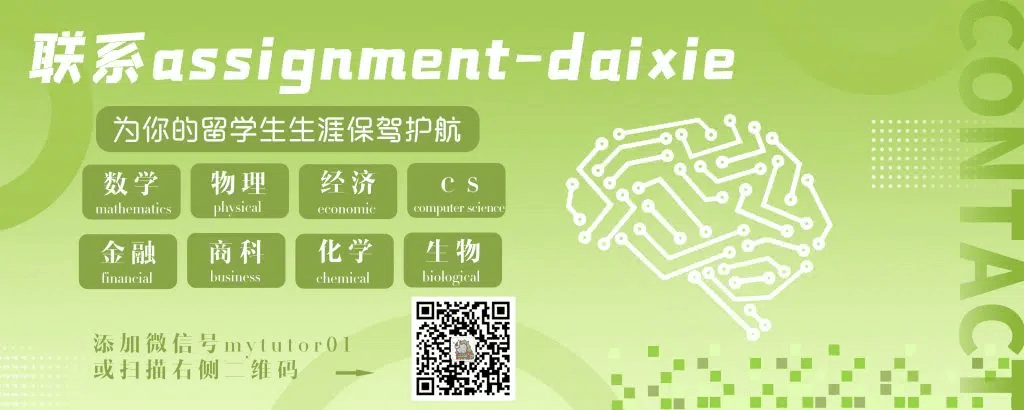