这是一份uwa西澳大学STAT3064的成功案例
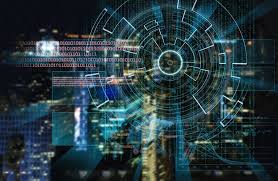
统计学习|STAT3064 Statistical Learning代写 UWA代写
$c_{0} \beta_{0}+c_{1} \beta_{1}$, hence on $g(x)=\beta_{0}+\beta_{1} x$. Since $C=R_{2}, V_{1}=V=\mathscr{L}(\mathbf{J}, \mathbf{x})$. Thus, $100 \%$ simultaneous confidence intervals on $g(x)=\beta_{0}+\beta_{1} x$ are given by
$$
\hat{g}(x) \pm K S\left(\hat{\eta}{c}\right) \quad \text { for } K=\sqrt{2 F{2, n-2, \gamma}}
$$
where $\hat{g}(x)=\hat{\beta}{0}+\hat{\beta}{1} x$. Since $\operatorname{Var}(g(x))=h(x) \sigma^{2}$, where $h(x)=1 / n+(x-\bar{x})^{2} /$ $S_{x x}$, the simultaneous intervals are
$$
\hat{g}(x) \pm\left[h(x) S^{2}\right]^{1 / 2} K
$$
We earlier found that a $100 \% \%$ confidence interval on $g(x)$, holding for that $x$ only, is
$$
g(x) \pm t h(x)^{1 / 2} S \quad \text { for } \quad t=t_{n-2,(t+n) 2}
$$
Thus the ratio of the length of the simultaneous interval at $x$ to the individual interval is $(K / t)=\left(2 F, 2 t^{2}-3(1+w /)^{1 / 2}\right.$, which always exceeds one.
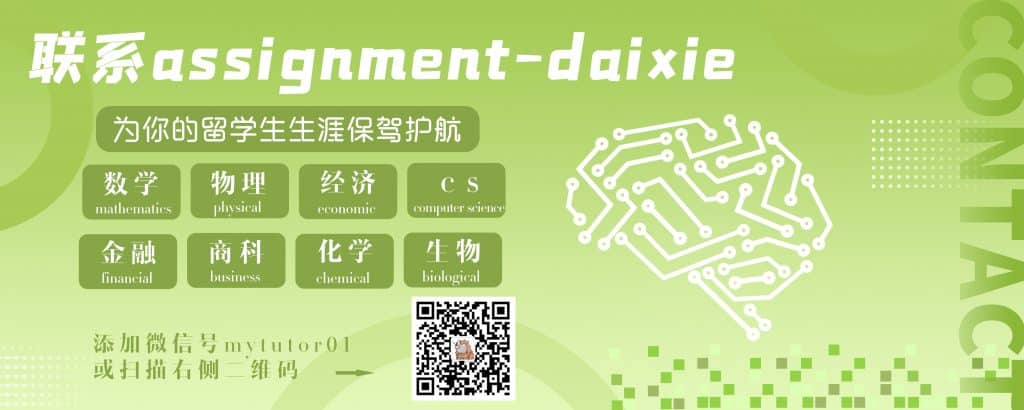
STAT3064 COURSE NOTES :
$$
\begin{aligned}
\beta_{j}=\bar{\mu}{\cdot j}-\mu=\frac{1}{I} \sum{i} \mu_{i j}-\mu \
(\alpha \beta){i j} &=\mu{i j}-\left[\mu+\alpha_{i}+\beta_{j}\right] .
\end{aligned}
$$
Then $\mu_{i j}=\mu+\alpha_{i}+\beta_{j}+(\alpha \beta){i j}$. The full model then can be written as follows. $$ \text { Full model: } \quad Y{i j k}=\mu+\alpha_{i}+\beta_{j}+(\alpha \beta){i j}+\varepsilon{i j k},
$$
where
$$
\sum_{1}^{l} \alpha_{i}=\sum_{1}^{J} \beta_{j}=\sum_{i}(\alpha \beta){i j}=\sum{j}(\alpha \beta){i j}=0, \quad \text { and } \quad \varepsilon{i j k} \sim N\left(0, \sigma^{2}\right)
$$