Assignment-daixieTM为您提供利物浦大学University of Liverpool STATISTICS & DATA ANALYSIS FOR ECONOMICS AND BUSINESS ECON112经济学和商业的统计和数据分析代写代考和辅导服务!
Instructions:
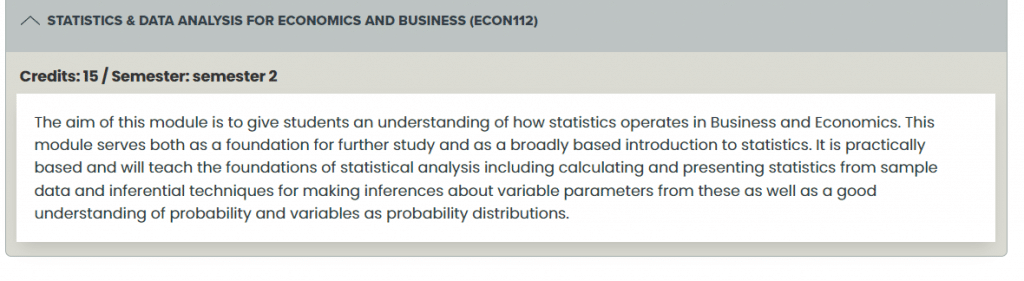
Suppose that the random variables $Y_1, \ldots, Y_n$ satisfy $$ y_i=\beta x_i+e_i, \quad i=1, \ldots, n $$ where $x_1, \ldots, x_n$ are fixed constants and $e_1, \ldots, e_n$ are i.i.d. normals with mean 0 and unknown variance $\sigma^2$. Assume that the hypothesis of interest is $H_0: \beta=0$. (a) Write the likelihood function (treating both $\beta$ and $\sigma^2$ as unknown). Write down score and information matrix.
The likelihood function is given by \begin{align*} L(\beta,\sigma^2) &= \prod_{i=1}^n \frac{1}{\sqrt{2\pi \sigma^2}}\exp\left(-\frac{(y_i – \beta x_i)^2}{2\sigma^2}\right) \ &= \frac{1}{(2\pi \sigma^2)^{n/2}}\exp\left(-\frac{1}{2\sigma^2}\sum_{i=1}^n(y_i – \beta x_i)^2\right) \end{align*}
The score function is given by
$\frac{\partial \ln L}{\partial \beta}=\frac{1}{\sigma^2} \sum_{i=1}^n x_i\left(y_i-\beta x_i\right)$
The information matrix is given by
$I\left(\beta, \sigma^2\right)=-\frac{\partial^2 \ln L}{\partial \beta^2}=\frac{n}{\sigma^2}$
(b) Find the unrestricted maximum likelihood estimator. Write the Wald test for the null hypothesis.
(b) To find the unrestricted maximum likelihood estimator, we need to maximize the likelihood function with respect to both $\beta$ and $\sigma^2$. Taking the partial derivatives of the log-likelihood function with respect to $\beta$ and $\sigma^2$, respectively, we obtain \begin{align*} \frac{\partial \ln L}{\partial \beta} &= \frac{1}{\sigma^2}\sum_{i=1}^n x_i(y_i – \beta x_i) = 0 \ \frac{\partial \ln L}{\partial \sigma^2} &= -\frac{n}{2\sigma^2} + \frac{1}{2\sigma^4}\sum_{i=1}^n(y_i – \beta x_i)^2 = 0 \end{align*}
Solving the first equation for $\beta$, we get
$\hat{\beta}=\frac{\sum_{i=1}^n x_i y_i}{\sum_{i=1}^n x_i^2}$
Substituting $\hat{\beta}$ into the second equation and solving for $\sigma^2$, we get
$\hat{\sigma}^2=\frac{1}{n} \sum_{i=1}^n\left(y_i-\hat{\beta} x_i\right)^2$
The Wald test for the null hypothesis $H_0: \beta=0$ is given by
$W=\frac{\hat{\beta}}{\sqrt{\widehat{\operatorname{Var}}(\hat{\beta})}} \sim \mathcal{N}(0,1)$
where $\widehat{\text{Var}}(\hat{\beta})$ is the estimated variance of $\hat{\beta}$, which is given by
$\widehat{\operatorname{Var}}(\hat{\beta})=\frac{\hat{\sigma}^2}{\sum_{i=1}^n x_i^2}$
(c) Solve the restricted maximization problem. Write the Lagrange Multiplier test.
To solve the restricted maximization problem, we need to maximize the restricted likelihood function under the null hypothesis $\beta=0$. The restricted likelihood function is obtained by substituting $\beta=0$ in the likelihood function:
$L\left(\sigma^2\right)=\prod_{i=1}^n \frac{1}{\sqrt{2 \pi \sigma^2}} \exp \left(-\frac{y_i^2}{2 \sigma^2}\right)=\frac{1}{\left(2 \pi \sigma^2\right)^{n / 2}} \exp \left(-\frac{1}{2 \sigma^2} \sum_{i=1}^n y_i^2\right)$
To maximize $L(\sigma^2)$ with respect to $\sigma^2$, we take the derivative with respect to $\sigma^2$ and set it equal to zero:
$\frac{\partial L\left(\sigma^2\right)}{\partial \sigma^2}=-\frac{n}{2 \sigma^2}+\frac{1}{2 \sigma^4} \sum_{i=1}^n y_i^2=0$
Solving for $\sigma^2$, we obtain:
$\hat{\sigma}^2=\frac{1}{n} \sum_{i=1}^n y_i^2$
This is the restricted maximum likelihood estimator of $\sigma^2$ under the null hypothesis $\beta=0$.
The Lagrange Multiplier test involves constructing a test statistic based on the difference between the unrestricted maximum likelihood estimator of $\sigma^2$ and the restricted maximum likelihood estimator of $\sigma^2$ under the null hypothesis $\beta=0$. The test statistic is given by:
$L M=\frac{\left(\hat{\sigma}{\text {unrestricted }}^2-\hat{\sigma}{\text {restricted }}^2\right) n}{\hat{\sigma}_{\text {uurestricted }}^2}$
where $\hat{\sigma}{\text{unrestricted}}^2=\frac{1}{n}\sum{i=1}^n (y_i-\hat{\beta}x_i)^2$ is the unrestricted maximum likelihood estimator of $\sigma^2$ and $\hat{\beta}$ is the maximum likelihood estimator of $\beta$.
Under the null hypothesis, the test statistic $LM$ follows a $\chi^2$ distribution with 1 degree of freedom. We reject the null hypothesis at level $\alpha$ if $LM>\chi_{1,\alpha}^2$, where $\chi_{1,\alpha}^2$ is the $(1-\alpha)$-quantile of the $\chi^2$ distribution with 1 degree of freedom.
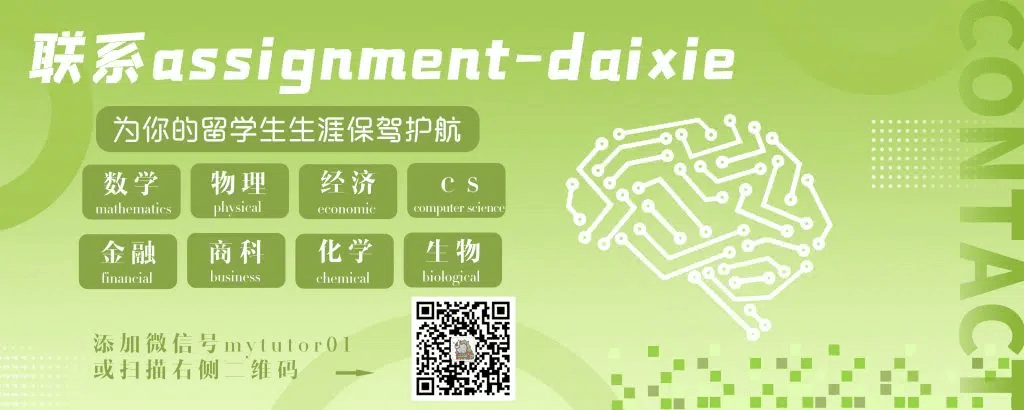