这是一份warwick华威大学ST118-15/ST119-10 的成功案例
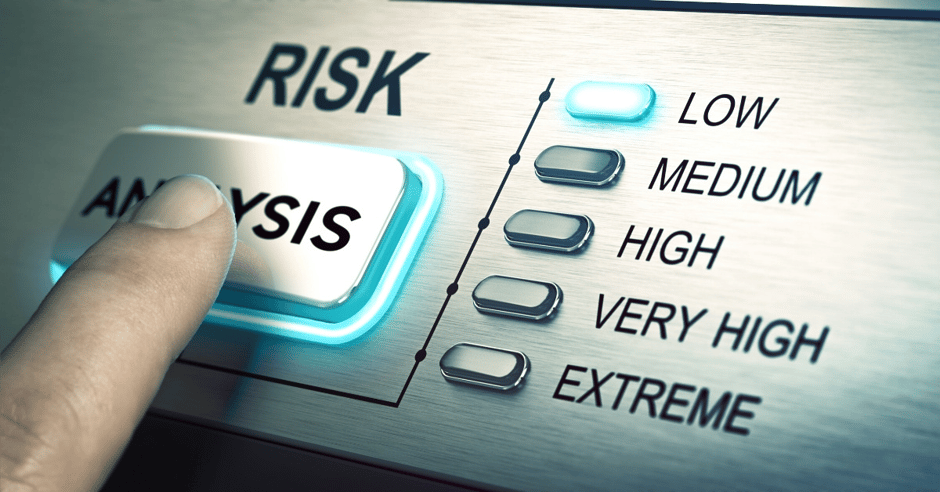
and it follows that when $X$ and $Y$ are independent
$$
w\left(u_{1}, u_{2}\right)= \begin{cases}\frac{1}{2 \pi \sigma^{2}} \frac{2 u_{1}}{1+u_{2}^{2}} e^{-u_{1}^{2} / 2 \sigma^{2}}, & u_{1}>0,-\infty0 \ 0, & u_{1} \leq 0\end{cases}
$$
and
$$
w_{2}\left(u_{2}\right)=\frac{1}{\pi\left(1+u_{2}^{2}\right)}, \quad-\infty<u_{2}<\infty
$$
respectively.
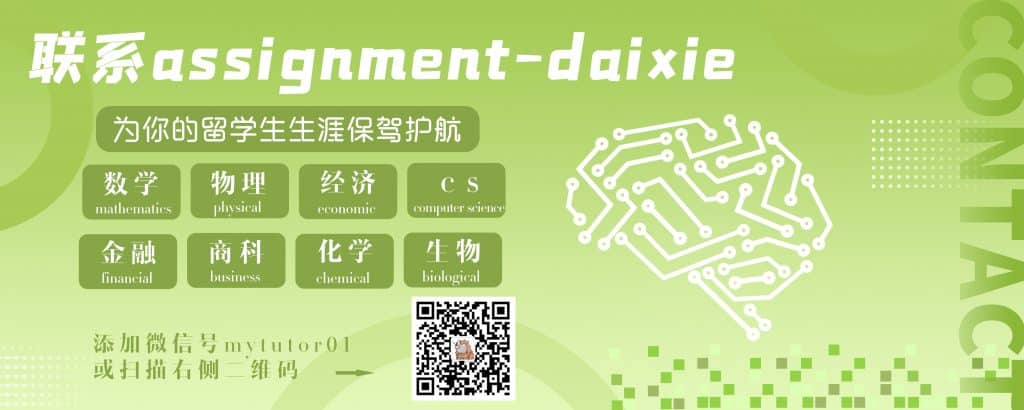
ST118-15/ST119-10 COURSE NOTES :
Let $F$ and $G$ be two absolutely continuous DFs; then
$$
H(x)=\int_{-\infty}^{\infty} F(x-y) G^{\prime}(y) d y=\int_{-\infty}^{\infty} G(x-y) F^{\prime}(y) d y
$$
is also an absolutely continuous DF with PDF
$$
H^{\prime}(x)=\int_{-\infty}^{\infty} F^{\prime}(x-y) G^{\prime}(y) d y=\int_{-\infty}^{\infty} G^{\prime}(x-y) F^{\prime}(y) d y
$$
If
$$
F(x)=\sum_{k} p_{k} \varepsilon\left(x-x_{k}\right) \quad \text { and } \quad G(x)=\sum_{j} q_{j} \varepsilon\left(x-y_{j}\right)
$$
are two DFs, then
$$
H(x)=\sum_{k} \sum_{j} p_{k} q_{j} \varepsilon\left(x-x_{k}-y_{j}\right)
$$