这是一份warwick华威大学MA251-12/MA249-12的成功案例
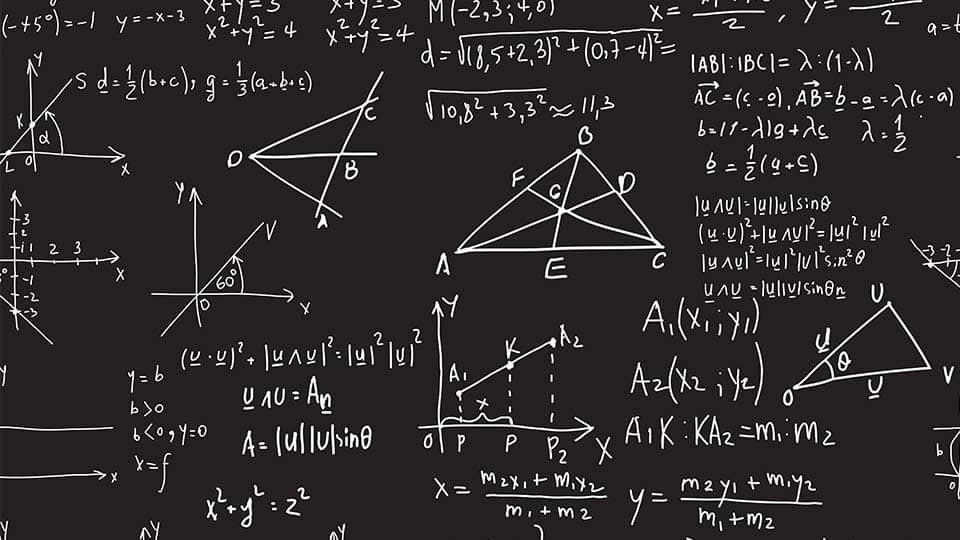
Let $A \in \mathbb{C}^{m \times n}$. A yields
(a) $\sigma_{i}\left(A^{T}\right)=\sigma_{i}\left(A^{}\right)=\sigma_{i}(\bar{A})=\sigma_{i}(A)$, for $i=1,2, \ldots, q$. (b) Let $k=\operatorname{rank}(A)$. Then $\sigma_{i}\left(A^{\dagger}\right)=\sigma_{k-i+1}^{-1}(A)$ for $i=1, \ldots, k$, and $\sigma_{i}\left(A^{\dagger}\right)=0$ for $i=$ $k+1, \ldots, q$. In particular, if $m=n$ and $A$ is invertible, then $$ \sigma_{i}\left(A^{-1}\right)=\sigma_{n-i+1}^{-1}(A), \quad i=1, \ldots, n . $$ (c) For any $j \in \mathbb{N}$ $$ \begin{gathered} \sigma_{i}\left(\left(A^{} A\right)^{j}\right)=\sigma_{i}^{2 j}(A), \quad i=1, \ldots, q ; \
\sigma_{i}\left(\left(A^{} A\right)^{j} A^{}\right)=\sigma_{i}\left(A\left(A^{*} A\right)^{j}\right)=\sigma_{i}^{2 j+1}(A) \quad i=1, \ldots, q .
\end{gathered}
$$
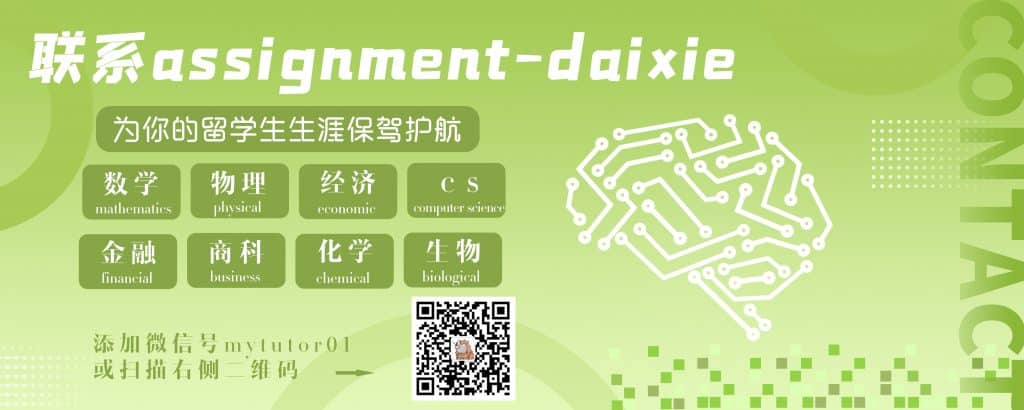
MA251-12/MA249-12 COURSE NOTES :
(Submatrices) Take $A \in \mathbb{C}^{m \times n}$ and let $B$ denote $A$ with one of its rows or columns deleted. Then $\sigma_{i+1}(A) \leq \sigma_{i}(B) \leq \sigma_{i}(A), \quad i=1, \ldots, q-1 .$
Take $A \in \mathbb{C}^{m \times n}$ and let $B$ be $A$ with a row and a column deleted. Then
$$
\sigma_{\mathrm{i}+2}(A) \leq \sigma_{\mathrm{i}}(B) \leq \sigma_{\mathrm{i}}(A), \quad i=1, \ldots, q-2 .
$$
The $i+2$ cannot be replaced by $i+1$. (Example 2 )
Take $A \in \mathbb{C}^{m \times n}$ and let $B$ be an $(m-k) \times(n-l)$ submatrix of $A$. Then
$$
\sigma_{i+k+l}(A) \leq \sigma_{i}(B) \leq \sigma_{i}(A), \quad i=1, \ldots, q-(k+l)
$$
Take $A \in \mathbb{C}^{m \times n}$ and let $B$ be $A$ with some of its rows and/or columns set to zero. Then $\sigma_{i}(B) \leq$ $\sigma_{i}(A), \quad i=1, \ldots, q$.
Let $B$ be a pinching of $A$. Then $\operatorname{sv}(B) \preceq_{w} \operatorname{sv}(A)$. The inequalities $\prod_{i=1}^{k} \sigma_{i}(B) \leq \prod_{i=1}^{k} \sigma_{i}(A)$ and $\sigma_{k}(B) \leq \sigma_{k}(A)$ are not necessarily true for $k>1$.